Comprehensive Guide to Capacity Modeling in Excel
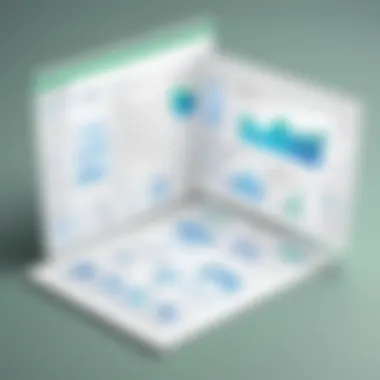
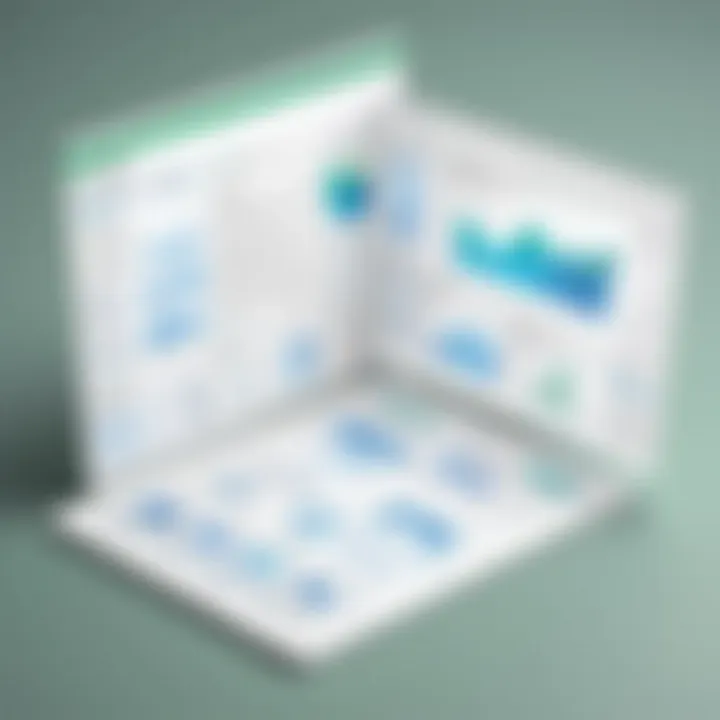
Intro
Capacity modeling is a crucial aspect of business operations, encompassing various methodologies aimed at optimizing resource use. In this guide, we will examine the ins and outs of capacity modeling specifically through Microsoft Excel. The relevance of mastering this skill cannot be overstated; it allows businesses to make informed decisions that can significantly enhance productivity and efficiency.
Excel is more than just a spreadsheet tool; it is a powerful platform capable of handling complex data analysis. Its functionalities are perfectly suited for capacity modeling, allowing professionals to plan accordingly, allocate resources efficiently, and respond to changing demands in real time. By the end of this guide, readers will gain not only theoretical understanding but also practical applications of capacity modeling techniques, focusing on best practices and common challenges.
Software Category Overview
Definition and Importance
Capacity modeling in Excel refers to the process of predicting the capacity needed to meet demand over a certain period. This predictive analysis is essential for maintaining optimal operations in any organization. Understanding how to model capacity can vastly improve decision-making processes, helping organizations to avoid the pitfalls of over-utilization and under-utilization of resources.
The importance of capacity modeling extends beyond mere projections. It allows organizations to anticipate future needs and trends, enabling them to adapt more fluidly to market demands and changing business landscapes. In today’s fast-paced environment, the ability to make data-driven decisions is more critical than ever.
Key Characteristics
A few key characteristics define effective capacity modeling:
- Data-Driven Analysis: Capacity modeling relies heavily on data. Effective models use historical data to forecast future requirements.
- Flexibility: The best models allow users to adjust variables dynamically, helping them stay relevant even as conditions change.
- Visual Representation: Graphs and charts within Excel can be invaluable for illustrating trends and patterns, making complex data comprehensible.
Comparative Analysis of Top Software Options
Feature Comparison
When considering Excel versus other software options for capacity modeling, it is essential to evaluate their features. While there are many tools available, Excel provides a balanced mix of simplicity, flexibility, and power for a diverse range of modeling needs. Key features include:
- Tables and PivotTables: Useful for summarizing large datasets and facilitating analysis.
- Formulas and Functions: Various built-in formulas can help with complex calculations without external tools.
- Visualization Tools: Charts and graphs help in visualizing capacity requirements succinctly.
Pricing Models
Excel's pricing is generally more approachable compared to specialized software. A subscription to Microsoft 365 includes access to Excel along with other applications. In contrast, advanced capacity modeling software like @Risk or AnyLogic often comes with substantial licensing fees, making Excel a competitive choice for budget-constrained organizations.
According to recent surveys, over 80% of small to medium-sized enterprises prefer using Excel for their analytical needs, owing to its versatility and low cost.
In summary, this guide will cover further intricacies of capacity modeling in Excel, providing readers with a roadmap to mastering this essential skill.
Prolusion to Capacity Modeling
Capacity modeling is a crucial aspect within the realm of business analysis. It refers to the process of assessing and optimizing the resources required to meet the demands of production or service delivery. In today’s fast-paced business environment, understanding how to effectively model capacity can significantly influence decision-making processes. It enables organizations to understand their operational capabilities and identify areas for improvement.
Defining Capacity Modeling
Capacity modeling is fundamentally concerned with determining how much of a product or service an organization can produce under specific conditions. It connects various aspects of operations, such as equipment availability, workforce productivity, and supply chain logistics. The main goal is to create a reliable template reflecting the maximum output potential over a certain period. This template can take multiple forms, whether visualizations in Excel or structured data sets prepared for analysis.
Importance in Business Analysis
Capacity modeling is important for several reasons. First, it aids in resource allocation. By understanding capacity limits, organizations can ensure that they are making the best use of their available resources. This allows for better planning, which reduces waste and enhances efficiency.
Additionally, capacity modeling provides critical insights during business forecasting. Companies can optimize their production schedules to align with anticipated demand, minimizing downtime and increasing responsiveness.
Finally, effective capacity modeling allows organizations to create more informed strategic decisions regarding investments in infrastructure or technology. A well-structured model can reveal the potential return on investment from increasing capacity through new equipment or technology implementation.
Excel as a Tool for Capacity Modeling
Excel is regarded as one of the most accessible tools for capacity modeling. Its versatility allows users to create models that can simulate different scenarios and variables. Excel’s functionalities, such as formulas, pivot tables, and charts, enable users to visualize and analyze data efficiently. Furthermore, the accessibility of Excel means that many professionals already possess a basic understanding of it, making it an ideal platform for capacity modeling tasks.
Utilizing Excel for modeling can facilitate collaborative efforts across teams. This flexibility is important, especially when various stakeholders contribute to the overall capacity strategy. While Excel may not offer the sophistication of more specialized software, its wide-ranging capabilities and ease of use make it a popular choice in many organizations.
"Capacity modeling in Excel is both practical and powerful, allowing businesses to optimize their resources effectively."
Core Concepts of Capacity Modeling
Understanding the core concepts of capacity modeling is crucial for professionals aiming to optimize processes and resources. This section discusses essential elements that form the foundation of capacity modeling, such as types of capacity and key performance indicators. Each of these components plays a significant role in guiding businesses through capacity planning. By grasping these principles, decision-makers can make more informed choices, thereby enhancing efficiency and productivity in their operations.
Types of Capacity
Capacity is not a uniform idea; it can be classified into three main types, reflecting different aspects of operational capability. These categories include Design Capacity, Effective Capacity, and Actual Capacity. Each one provides unique insights into how well a business can utilize its resources.
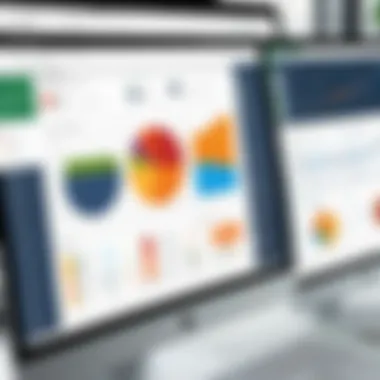
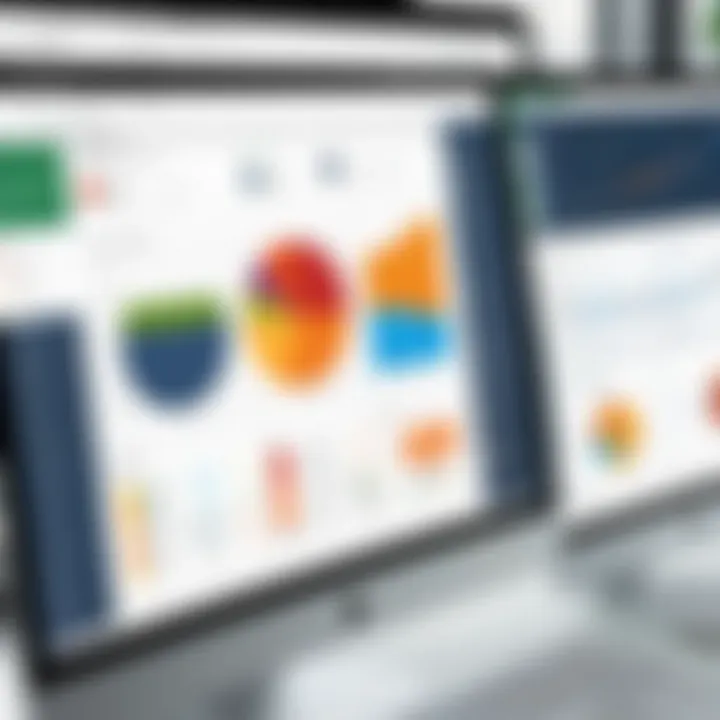
Design Capacity
Design Capacity refers to the maximum output that a system or process can achieve under ideal conditions. This metric is vital because it sets a benchmark for what the system is theoretically capable of producing. One key characteristic of Design Capacity is that it assumes optimal functioning without any interruptions. This makes it a beneficial reference point for understanding maximum potential. However, the drawback lies in its idealistic nature. It does not necessarily account for real-world variables like downtime or operational inefficiencies that may significantly reduce actual outputs.
Effective Capacity
Effective Capacity takes into account the real-world conditions and factors that limit production. This metric represents the output a system can achieve under normal, everyday operating conditions. What distinguishes Effective Capacity is its practicality; it is a more realistic figure compared to Design Capacity. By using Effective Capacity, businesses can set more achievable production goals. The downside is that while it is more realistic, it may not fully account for every possible limitation that could arise, such as unforeseen machinery breakdowns.
Actual Capacity
Actual Capacity is the metric that reflects true performance. It measures the actual output produced during a specific period. This is a critical aspect because it provides a complete picture of operational efficiency. The key characteristic of Actual Capacity is that it is based on historical data and reflects the day-to-day reality of production. It is highly beneficial as it allows businesses to analyze performance against both Design and Effective Capacities. However, the challenge with using Actual Capacity is that it can sometimes lead to overly negative assessments if not compared against appropriate benchmarks, like Effective Capacity.
Key Performance Indicators
Key Performance Indicators (KPIs) are essential measurement tools that help track performance related to capacity modeling. They provide actionable data that can trigger timely interventions and adjustments. The three primary KPIs discussed here are Utilization Rates, Throughput, and Bottleneck Analysis.
Utilization Rates
Utilization Rates define the extent to which a business utilizes its available capacity. This measurement is significant because it can highlight inefficiencies or areas for improvement. The essential aspect of Utilization Rates is that it provides direct feedback on resource allocation. A high utilization rate often indicates that resources are being utilized effectively, which is advantageous for businesses focused on maximizing output. However, excessively high rates may signal overloading, risking quality and employee burnout if persistent.
Throughput
Throughput measures the rate at which products are completed and ready for delivery. It reflects the efficient flow of production in a system. Understanding Throughput helps in identifying production capacity and ensuring timely fulfillment of demand. A key advantage of monitoring Throughput is its ability to shed light on the hidden inefficiencies within a production line. However, relying too much solely on Throughput can lead to ignoring other critical variables like quality control and maintenance.
Bottleneck Analysis
Bottleneck Analysis focuses on identifying the points in a process that slow down overall performance. By finding and addressing these constraints, businesses can enhance overall capacity. The key characteristic of Bottleneck Analysis is that it helps reveal systemic issues that may not be visible at first glance, enabling targeted improvements. While this method is powerful in identifying weaknesses, one challenge is ensuring that improvements made do not inadvertently create new bottlenecks elsewhere in the system.
Understanding these core concepts in capacity modeling can significantly enhance a company's ability to optimize resources and improve decision-making.
Setting Up Excel for Capacity Modeling
Establishing a strong foundation in Excel for capacity modeling is vital for effective analysis and decision-making. The right setup allows professionals to manipulate data efficiently, visualize trends, and draw actionable insights. Understanding how to leverage Excel's functionalities can lead to enhanced accuracy and better outcomes in capacity planning.
Choosing the Right Template
Selecting an appropriate template is a key step in setting up your capacity model in Excel. A good template can streamline your workflow, standardize calculations, and serve as a solid framework for analysis. Templates often come pre-formatted for specific capacity-related tasks, which reduces the time spent on initial setup.
Ensure that the template aligns with your objectives. For instance, if you need to model manufacturing capacity, look for templates tailored for production data. This choice not only saves time but also minimizes errors during data entry.
Data Input and Structure
Organizing Data for Analysis
Organizing data effectively is crucial for clarity and usability in capacity models. A well-structured spreadsheet makes it easier to analyze trends and evaluate performance metrics. For example, separate sheets for raw data, calculations, and results can significantly improve navigation. This organization helps in pinpointing where specific information resides. It also enhances collaboration, as different stakeholders can focus on their respective areas without confusion.
A notable characteristic of organizing data is consistency. Using consistent formats (like date formats or unit measurements) is important to avoid misunderstandings. Furthermore, when the data is organized, it becomes easier to apply various Excel functions without running into compatibility issues. This kind of organization is a popular choice for a clear and methodical approach.
Using Tables for Clarity
Utilizing tables in Excel provides a clearer view of the data and can enhance its readability. Tables allow for easy sorting and filtering, enabling faster data analysis. They automatically expand to accommodate additional data, which eliminates one potential pitfall of using standard ranges in Excel.
The unique feature of tables is their ability to create structured references. This makes it easier to write formulas that automatically adjust to changes in the data set. Conversely, one drawback can be that tables, when misused, might complicate simple data sets, making them unnecessarily complex.
Key Formulas for Capacity Modeling
Understanding key Excel formulas is essential for effective capacity modeling. These formulas enable robust data manipulation and insightful analysis.
IF Statements
IF statements are fundamental in capacity modeling as they allow for conditional calculations. This capability is crucial when dealing with varying scenarios, such as determining whether a specific capacity meets predetermined thresholds. The versatility of the IF function makes it a go-to option for many models.
One key characteristic of IF statements is their straightforward logic; they allow users to define outcomes based on specific conditions. However, keeping them simple is important, as overly complex IF statements can lead to confusion.
SUMIFS and AVERAGEIFS
SUMIFS and AVERAGEIFS are essential for summarizing data under multiple criteria. These functions not only add or average numbers but do so based on selected conditions, which is crucial in capacity analysis. For instance, calculating average usage levels under certain constraints helps in understanding resource allocation.
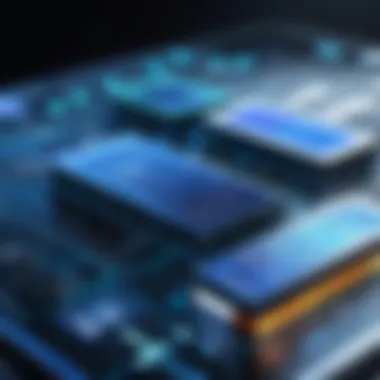
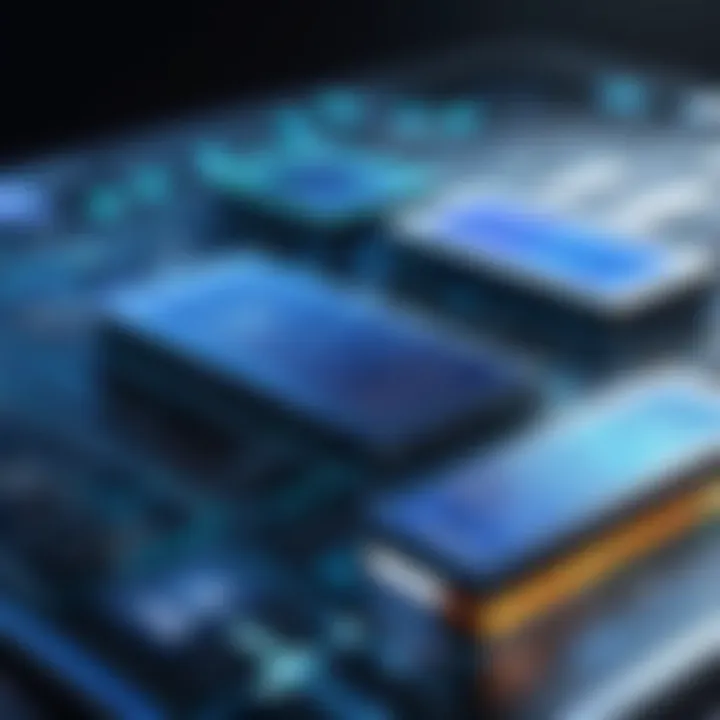
Their key characteristic is the ability to analyze large datasets effectively. This functionality is especially beneficial for capacity modeling as it reduces manual calculations and errors. However, users must ensure that their data is clean to avoid inaccuracies in results.
INDEX and MATCH Functions
The INDEX and MATCH functions allow for dynamic data retrieval, offering a more flexible alternative to traditional VLOOKUP. By combining these functions, users can locate specific values in datasets efficiently. This capability is particularly useful in complex models where data is spread across multiple sheets or tables.
The flexibility of INDEX and MATCH contributes significantly to the analytical depth of capacity models. A potential drawback is the initial learning curve associated with mastering these functions; however, once understood, they can greatly enhance a model's functionality.
Analyzing Capacity with Excel
Analyzing capacity using Excel is a pivotal step in effective capacity modeling. This process allows businesses to evaluate their operational capabilities while making informed decisions. By examining different variables, organizations can identify potential bottlenecks and optimize their resource allocation. Excel serves as a flexible tool that can handle complex data sets, enabling better analysis and visualization of capacity metrics. Understanding how to effectively analyze capacity in Excel helps professionals develop a more strategic approach to their operations.
Scenario Analysis
Creating What-If Scenarios
Creating what-if scenarios is critical for decision-making processes. This method allows users to explore various outcomes based on changing inputs. By adjusting assumptions in their models, users can visualize the impact on capacity. This approach is particularly useful when evaluating different business strategies or operational changes. A key characteristic of what-if analysis is its simplicity; it can easily integrate into existing Excel spreadsheets.
One unique feature is the ability to create multiple scenarios. Users can compare best-case, worst-case, and expected outcomes side by side. This versatility helps organizations plan proactive strategies and identify risks. However, reliance on this analysis requires careful consideration of the inputs. If the foundation data is flawed, the scenarios may lead to misleading conclusions.
Using Data Tables for Sensitivity Analysis
Using data tables for sensitivity analysis enhances understanding of how varying inputs affects outcomes. This tool allows users to assess how changes in key variables influence capacity projections. It is especially beneficial in analyzing scenarios with multiple variables, providing a visual representation of potential outcomes. The main advantage of data tables is that they can quickly summarize extensive data sets in a clean format.
A unique feature of data tables is their ability to connect multiple scenarios into a single visual. This capability makes it easier for decision-makers to determine the most favorable conditions. Nonetheless, users must be mindful of complexity. Overly intricate models with too many variables can hinder clear analysis and may lead to confusion rather than clarity.
Visualization Techniques
Creating Charts for Capacity Insights
Creating charts for capacity insights greatly enhances the communication of data. Visual representations simplify complex information, making it more accessible to decision-makers. Well-designed charts can reveal trends and patterns that may not be immediately evident in raw data. A key characteristic of charts is that they allow for quick evaluation of performance ratios, offering a snapshot of operational efficiency.
One unique aspect of charts is their capacity to highlight critical data points. For instance, pie charts can illustrate proportional capacity distributions, while bar charts can compare performance metrics over different periods. However, care must be taken not to overcomplicate the charts. Simple, clear visuals tend to be more effective for conveying important insights without overwhelming the audience.
Utilizing Conditional Formatting
Utilizing conditional formatting is a powerful method to enhance data readability. This technique allows users to set visual alerts based on predefined conditions, drawing attention to critical values. For example, high utilization rates can be highlighted in red, while lower rates might be displayed in green. This feature promotes quicker interpretation of data, supporting faster decision-making.
The key feature of conditional formatting is its flexibility. Users can apply it across tables and graphs, ensuring that all relevant data is easy to interpret at a glance. Although highly beneficial, there is a risk of overuse. Excessive formatting can lead to confusion and can detract from the data's clarity, making it vital to use it judiciously.
Best Practices in Capacity Modeling
In the world of capacity modeling, utilizing best practices is crucial for achieving optimal outcomes. These practices guide professionals in creating models that are not only efficient but also accurate and reliable. The importance of adhering to these principles becomes evident, particularly in complex business environments where data-driven decision-making is essential. A robust capacity model can lead to significant operational improvements, cost reductions, and enhanced forecasting capabilities.
Regular Updates and Maintenance
Regular updates and maintenance form the backbone of any effective capacity modeling approach. As business conditions evolve, so do the underlying data and assumptions that inform capacity models. It is vital for analysts to frequently review and refresh the data to ensure that the model reflects the latest trends and insights. This practice not only enhances the accuracy of the findings but also instills confidence among stakeholders.
To facilitate this process, companies can implement automated systems to update data inputs. This allows for real-time monitoring and reduces the risk of human error. In addition, maintenance routines should include revisiting the model structure and formulas to ensure continued relevance and reliability. Without regular updates, the model may grow stale, leading to poor decisions based on outdated or irrelevant information.
Collaborative Modeling Approaches
Collaboration in capacity modeling can significantly enhance the quality of the analyses. Engaging teams from various departments—such as finance, operations, and IT—ensures that a diverse range of expertise informs the model. This collaborative approach fosters a holistic view of the project scope, which can reveal insights that might be missed when working in silos.
Sharing and Version Control
One critical aspect of collaborative modeling is sharing and version control. This process allows multiple team members to work on the same model while keeping track of changes. Version control ensures that updates are documented and previous iterations are preserved, providing a safety net against data loss or inaccuracies.
The main characteristic of sharing and version control lies in its ability to streamline team efforts. It promotes transparency and accountability among members, making it easier to identify who made specific changes. This practice is especially beneficial in large-scale projects where numerous contributors are involved.
However, managing version control can be complex. A well-defined system is necessary to prevent confusion over file versions. Using platforms like Git or cloud storage solutions can help mitigate these challenges. The unique feature of these systems—the ability to merge changes from different users—enhances collaboration while preserving the integrity of the model.
Utilizing Comments for Clarity
Another valuable aspect of collaborative modeling is utilizing comments for clarity. In large Excel models, it can become challenging to track the reasoning behind specific formulas or data selections. By integrating comments directly into the spreadsheet, users can provide context and explanations for their choices.
This method enhances communication within the team, ensuring that all members can understand and follow the logic of the model. Moreover, comments can help onboard new team members more quickly. The key characteristic of utilizing comments is its straightforward implementation—existing Excel tools allow for easy insertion of notes without disrupting the flow of the model.
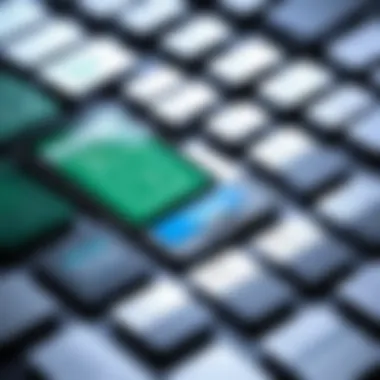
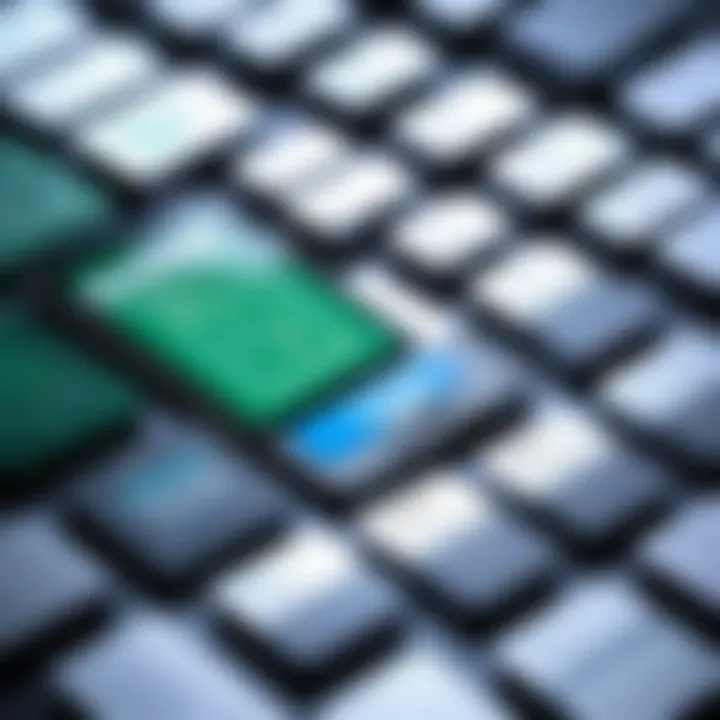
However, overusing comments may lead to clutter, reducing the model's usability. Striking a balance is essential; it is critical to ensure that comments are concise and relevant to avoid confusion. Additionally, pairing comments with structured documentation in a separate guide can provide a clearer overview without overwhelming the model itself.
"Effective capacity modeling is not just about numbers; it's about strategic collaboration and continuous improvement."
In summary, adopting best practices in capacity modeling paves the way for more accurate, reliable, and useful models. Regular updates and a collaborative approach ensure that these models serve their purpose in supporting informed decision-making.
Common Pitfalls and How to Avoid Them
Capacity modeling is a crucial process for predicting and managing the resources within an organization. However, there are several common pitfalls that can hinder effective capacity modeling. Addressing these issues is essential for creating reliable models that facilitate informed decision-making. Recognizing and avoiding these pitfalls can dramatically enhance the accuracy and utility of capacity planning efforts.
Overcomplicating Models
One major pitfall in capacity modeling is overcomplicating the model unnecessarily. As more variables and formulas are added, the model can become so complex that it loses clarity. This often leads decision-makers to misunderstand the results or misinterpret data. Keeping models simple and focused is key.
- Focus on Key Metrics: Determine which performance indicators are most relevant to your analysis and concentrate on them.
- Limit Inputs: Avoid excessive data points that do not contribute to insights. Stick to essential data that genuinely influences capacity decisions.
- Use Documentation: Include notes or comments within the Excel model, explaining the reason for specific calculations or choices.
Ignoring Data Quality
The quality of data used in capacity modeling is another critical factor. Poor data quality can result in unreliable outputs, leading to faulty decision-making. It is vital to ensure that the data is accurate and up-to-date.
- Validate Data Sources: Always confirm the reliability of data sources before using them in your models.
- Regular Checks: Implement routine checks to identify and correct discrepancies in data. Consistently monitor changes in data quality by reviewing data entry procedures.
- Use Data Cleaning Techniques: Incorporating methods such as de-duplication, normalization, and cross-validation can help maintain integrity in your data.
Neglecting User Training
Another common oversight is neglecting user training. Even the most sophisticated models will be ineffective if users are not adequately trained to interpret the data correctly or to use Excel functions proficiently. This training ensures users can utilize the model effectively and extract meaningful insights.
- Conduct Training Sessions: Organize workshops or training programs tailored to the complexities of your capacity model.
- Provide Resources: Offering guides, checklists, and resources can empower users to understand the model better.
- Encourage Feedback: Cultivate an environment where users can provide feedback on the model. This input can highlight areas needing further assistance or clarification.
Avoiding these common pitfalls in capacity modeling is essential. It preserves the model's integrity and fosters a more productive analytical environment. By keeping models straightforward, ensuring data quality, and investing in user training, organizations can improve their capacity planning outcomes.
Future Trends in Capacity Modeling
As the landscape of data management and decision-making continues to evolve, capacity modeling is not left untouched. Understanding future trends in this area is essential for businesses aiming for efficiency and performance. This section will explore critical advancements such as artificial intelligence integration, the rise of cloud solutions, and their implications for capacity modeling in Excel.
Integrating AI and Machine Learning
Artificial intelligence (AI) and machine learning (ML) are becoming increasingly relevant in capacity modeling. These technologies provide robust analytical capabilities that enhance decision-making processes. By automating data analysis, AI can quickly identify patterns and trends that may not be immediately obvious to human analysts.
Benefits of AI in Capacity Modeling
- Improved Accuracy: AI reduces human errors by applying complex algorithms to large data sets. This leads to more precise capacity forecasts.
- Predictive Analytics: Machine learning models can predict future demands based on historical data, allowing more effective resource allocation.
- Scenario Simulation: Advanced AI tools can simulate various scenarios, offering decision-makers insights into potential outcomes based on different variables.
Considering these factors, businesses must embrace AI as a tool to improve their capacity modeling. The implementation might require investment in training staff and tools, as well as adjusting current processes to fully exploit these capabilities.
Cloud Solutions and Accessibility
Cloud technology has transformed resource management by providing scalable solutions that can adjust to business needs rapidly. Capacity modeling is no exception. With cloud solutions, data can be more accessible and easier to manage. These solutions foster collaboration among team members, regardless of their physical location.
Advantages of Cloud Solutions
- Real-Time Collaboration: Team members can work on models simultaneously, ensuring that data is up-to-date and reducing the chance of miscommunication.
- Scalability: Organizations can easily adjust their storage and processing power as their needs change, allowing them to grow without the burdens of traditional hardware.
- Data Security: Leading cloud providers typically offer robust security measures to protect sensitive data, allowing organizations to trust their capacity models.
In summary, the integration of AI and cloud technologies in capacity modeling presents significant opportunities for improving efficiency and decision-making. Businesses that adapt to these trends will likely gain a competitive edge in their respective markets.
End
In this article, we have explored vital aspects of capacity modeling using Excel. The importance of effective capacity modeling cannot be overstated. It allows businesses to make informed decisions that enhance operational efficiency. The ability to predict resource requirements, identify potential bottlenecks, and optimize processes can significantly improve productivity.
Summarizing Key Insights
Throughout the article, a series of key insights have emerged that guide the capacity modeling process:
- Understand the core concepts: Knowing the different types of capacity—design, effective, and actual—assists in realistic modeling.
- Importance of KPIs: Utilizing key performance indicators such as utilization rates and bottleneck analysis is essential for monitoring capacity.
- Excel setup: Properly organizing data in Excel through effective formula application can streamline the modeling process.
- Visualization: Utilizing charts and conditional formatting fosters better analysis by providing clear insights into capacity scenarios.
- Best practices and pitfalls: Regular updates and collaborative approaches can maintain model relevance while avoiding common mistakes like data quality neglect.
These elements contribute to the synergy needed for maintaining operational efficacy and demand fulfillment.
Next Steps for Implementation
Moving forward, implementing a structured capacity modeling process requires several actions:
- Evaluate current processes: Assess existing operational workflows to identify areas where capacity modeling can provide benefits.
- Choose the right Excel template: Depending on organizational needs, selecting a suitable Excel template is a critical first step.
- Train staff: Ensuring that team members are familiar with Excel’s advanced functions will enhance modeling accuracy.
- Integrate insights into planning: Use data obtained from capacity models in strategic planning discussions.
- Monitor and revise: Regularly revisit models and update projections based on changing business landscapes or data alterations.
By following these steps, organizations can enhance their capacity planning strategy. Adapting and refining these processes will not only improve operational effectiveness but also drive better business outcomes.