Comprehensive Guide to Evaluating Predictive Analytics Software
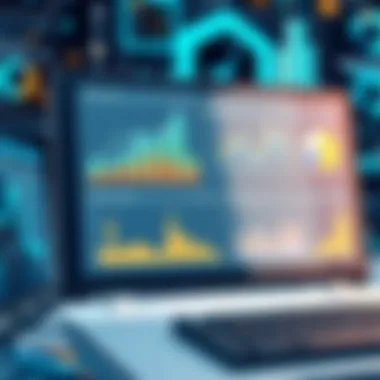
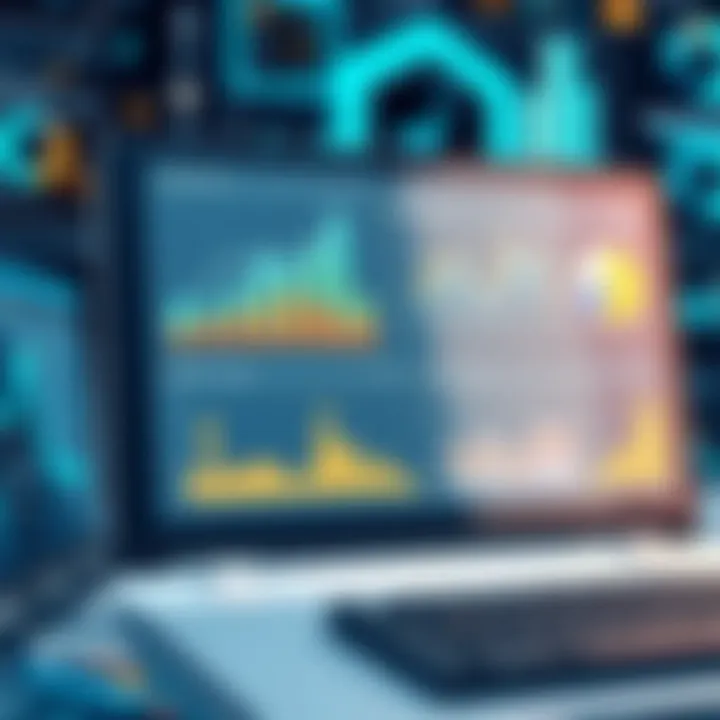
Intro
Predictive analytics software has become crucial in today’s data-driven world. For decision-makers and IT professionals, the ability to analyze upcoming trends and consumer behavior can mean the difference between staying ahead of the competition and becoming obsolete. This guide will explore how to evaluate various predictive analytics solutions, highlighting the features that really matter, analyzing aspects like pricing and applicability, and ultimately guiding users toward informed decisions.
The landscape of predictive analytics tools can be a bit like trying to find your way through a maze; it's easy to get lost amid the hype and marketing jargon. Therefore, understanding the scope of available software and its core functionalities is not just important; it's essential.
Software Category Overview
Definition and Importance
Predictive analytics software empowers businesses to forecast future outcomes based on past data patterns. Rather than merely reacting to trends, these tools provide actionable insights that can significantly shape strategic planning. The rise of big data has only amplified the significance of these tools, enabling organizations to hone in on their target audience, optimize their marketing strategies, and sharpen their competitive edge.
Many decision-makers realize that the importance of predictive analytics extends beyond just understanding customer preferences. It also supports risk management, enhances operational efficiency, and drives innovation.
Key Characteristics
When sifting through the myriad of options, certain features become essential:
- Data Integration: The ability to seamlessly gather and process data from various sources is non-negotiable. Software that supports diverse data formats enhances its versatility.
- User-Friendliness: A tool that requires an army of data scientists might not be practical for most organizations. Look for solutions that are intuitive enough for non-technical users while still offering robust capabilities.
- Scalability: As businesses grow, their data needs will evolve. Predictive analytics tools must be scalable to adapt to increasing data volumes without diminishing performance.
- Visualization Tools: Effective data visualization helps in interpreting complex data sets. The more user-friendly the visualization, the quicker insights can be drawn.
A powerful predictive analytics tool is like having a crystal ball that gives you foresight into future trends and behaviors. Without it, you might just be guessing in the dark.
Comparative Analysis of Top Software Options
Feature Comparison
Not all predictive analytics software is created equal. When evaluating options, it’s crucial to understand key features:
- Statistical Models: Some software leans heavily on machine learning models, while others might rely more on traditional statistical methods. Your choice will depend on the specific requirements of your project.
- Collaboration Capabilities: If multiple departments need access to analytics, finding software that facilitates easy sharing and collaboration can streamline workflows.
- Support & Training: Consider if the provider offers training sessions and ongoing support. This can be a game-changer when implementing complex analytical tools.
Pricing Models
When it comes to purchasing predictive analytics software, you’ll find various pricing models:
- Subscription-Based: This model requires ongoing payment but often includes updates and support.
- Pay-Per-Use: Ideal for businesses that may not need regular access, these plans can help cut costs.
- One-Time Purchase: Though initially expensive, this might be favorable for those looking for long-term use without ongoing costs.
In summary, evaluating predictive analytics software is a significant endeavor for any organization. By honing in on essential features, understanding varying pricing models, and recognizing the importance of scalability and user-friendliness, decision-makers can make more informed choices that will ultimately empower their strategic initiatives.
Understanding Predictive Analytics Software
Predictive analytics software focuses on using data, statistical algorithms, and machine learning techniques to identify the likelihood of future outcomes based on historical data. Understanding this software is crucial for organizations that aim to harness data for informed decision-making. The relevance of this topic stretches across various industries, where predictive analytics enhances operational performance, encourages proactive strategies, and facilitates improved risk assessment.
Definition and Purpose
At its core, predictive analytics seeks to forecast future events. This involves interpreting past behaviors to estimate what may occur down the line. Businesses leverage this software to anticipate trends, client needs, and potential risks. The key purpose is to make data-driven decisions that can enhance the efficiency of operations and communication across the board.
Key Components of Predictive Analytics
Delving deeper into predictive analytics, several components are pivotal in shaping its effectiveness. Each plays a unique role in constructing a robust framework for analysis and insight generation.
Data Collection
Data collection forms the foundation of predictive analytics. Without quality data, no reliable predictions can be made. It involves gathering relevant information from various sources, such as sales figures, customer feedback, and market trends. The quality and quantity of data collected directly influence the predictive accuracy.
- Key Characteristic: Comprehensive and high-quality datasets are vital for credible analytics results.
- Unique Feature: Some systems can pull in data in real-time, allowing for up-to-date insights.
- Advantages: Detailed data can enhance the granularity and context of predictions but can also lead to complexity if not managed well.
Modeling Techniques
Modeling techniques represent the methods used to analyze incoming data and make predictions. These might include regression analysis, decision trees, or neural networks. Each methodology comes with its strengths and potential drawbacks.
- Key Characteristic: Flexibility in choosing the modeling technique allows for tailored solutions to the specific challenges faced by a business.
- Unique Feature: Many modern platforms provide automated modeling options, which save time for the user.
- Disadvantages: Certain methodologies can be overfitted to past data, which may result in unreliable future forecasts.
Statistical Algorithms
Statistical algorithms are the mathematical foundations that power predictive models. They help in mimicking patterns from historical data to forecast future incidences. The choice of algorithm can significantly impact the output.
- Key Characteristic: Robust algorithms can handle large datasets without losing accuracy.
- Unique Feature: Advanced algorithms, such as ensemble methods, can combine different approaches to improve prediction reliability.
- Advantages and Disadvantages: While sophisticated algorithms can enhance accuracy, they may also require significant computational power and expertise to implement adequately.
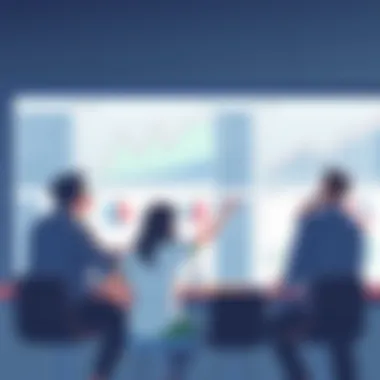
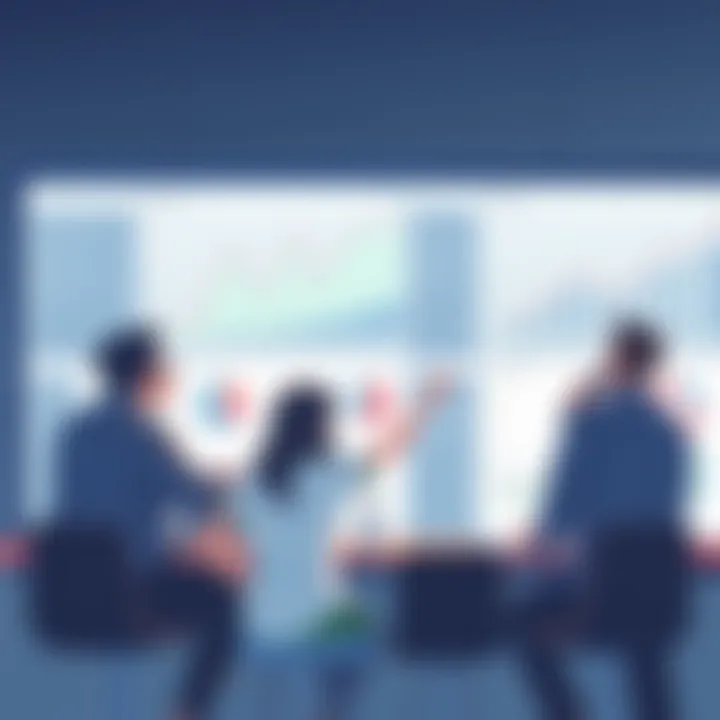
Benefits of Predictive Analytics
Understanding the main benefits of predictive analytics helps one appreciate its growing importance in data-driven decision-making. Its potential spans a wide array of applications and sectors.
Enhanced Decision-Making
Enhanced decision-making is one of the hallmark advantages of predictive analytics. By providing insights into possible futures, it empowers organizations to choose proactively rather than reactively. Well-informed decisions can lead to significant improvements in strategy and outcome.
- Key Characteristic: The capability to foresee market trends and customer behavior allows companies to tailor their strategies effectively.
- Unique Feature: Some solutions offer scenario analysis, allowing decision-makers to evaluate various outcomes before taking action.
- Advantages and Disadvantages: Although the insights gained can be invaluable, there is a danger of over-relying on predictions, neglecting the uncertainty involved in market dynamics.
Operational Efficiency
Operational efficiency is another benefit that organizations can reap from predictive analytics. By anticipating potential issues in workflows or supply chains, companies can optimize resources, streamline processes, and reduce waste.
- Key Characteristic: Efficiency-driven insights can lead to better allocation of budgets and human resources.
- Unique Feature: Predictive maintenance in manufacturing can preemptively address machinery failures, cutting costs down the line.
- Advantages and Disadvantages: The efficiency gains can be substantial, but they rely on the quality and integrity of the underlying data.
Risk Management
Predictive analytics significantly enhances risk management practices. By recognizing potential risks before they materialize, organizations can put in place mitigation strategies, staying one step ahead of any threats.
- Key Characteristic: Identifying risks through data patterns can promote a more proactive rather than reactive stance on risk management.
- Unique Feature: Some systems incorporate advanced analytics to predict fraud or cybersecurity threats.
- Advantages and Disadvantages: The anticipation of risk is beneficial, yet false positives or inaccuracies in the model can lead to unwarranted alarm or misallocation of resources.
In summary, a firm grasp of understanding predictive analytics software sets up decision-makers for success. Each component, from data collection to statistical algorithms, shapes the predictive landscape. With awareness of its benefits in decision-making, operational efficiency, and risk management, organizations can effectively steer their strategies towards future opportunities.
Criteria for Selecting Predictive Analytics Software
The selection of predictive analytics software is a pivotal step for organizations aiming to enhance their data-driven decision-making capabilities. With an increasing flow of data, companies are not only looking for software that processes information but rather for tools that provide actionable insights. Understanding the criteria for selecting the appropriate software can prove beneficial in streamlining operations and driving growth.
User-Friendly Interface
When choosing predictive analytics software, the user interface can make or break the deal. A complex layout may be off-putting, especially for those who are not data scientists. As such, a user-friendly interface is not merely a luxury; it’s a necessity. It allows stakeholders, regardless of their technical background, to engage with the data. Consider software options that offer intuitive dashboards, drag-and-drop functionalities, and clear visualizations to facilitate understanding.
Key takeaways include:
- Ease of Navigation: The interface should encourage easy exploration of data without requiring extensive training.
- Customization Options: Users could benefit from dashboards that can be tailored to their needs, ensuring relevant data is displayed.
- Responsive Design: As more professionals work remotely, having a mobile-friendly design is essential.
"An intuitive interface can turn data sceptics into data enthusiasts by making the insights more accessible."
Integration Capabilities
No software exists in a vacuum, and the ability of predictive analytics tools to integrate seamlessly with existing systems is crucial. Organizations often use a myriad of applications for different functions, from CRM to ERP systems. Therefore, the software should offer robust integration capabilities with ease; otherwise, siloed data can undermine the entire analytics effort.
Look for:
- Pre-built Connectors: These can save time during implementation, ensuring a smoother transition.
- API Availability: APIs allow for custom integrations, tailoring the software to meet specific operational needs.
- Data Import/Export Options: General compatibility with data formats can reduce friction in data usage across different platforms.
Scalability and Flexibility
As businesses evolve, their needs change. It’s essential for the predictive analytics software to not just serve current needs but also scale in response to growing requirements. Choosing software that is inherently flexible can save time and costs in the long run.
Consider the following aspects:
- Modular Architecture: This allows adding features or users without restructuring the entire setup.
- Performance on An Increased Data Volume: Test the software under varying loads to see how well it adapts to more data.
- Subscription Models: Opt for a pricing structure that aligns with business growth, where costs increase in parallel with utilization.
By focusing on user-friendliness, integration capabilities, and scalability, organizations can efficiently navigate the maze of predictive analytics software and select the best fit for their distinct needs.
Popular Predictive Analytics Software Options
When diving into the sea of predictive analytics software, it is crucial to identify which tools stand out. The right software can make a world of difference in how organizations interpret data, make forecasts, and ultimately drive their strategic decisions. Here, we’ll break down some popular options, highlighting their core functionalities, use cases, and client feedback. By scrutinizing these elements, decision-makers can better align these tools with their needs, paving the way for more effective analytics strategies.
Software A: Overview and Features
Core Functionalities
Software A boasts a range of core functionalities that are designed to cater specifically to predictive modeling. It emphasizes user-friendly dashboards and automated reporting, simplifying the analysis process for users at all levels. One of its standout features is the built-in data visualization tools, which allows users to convert raw data into intuitive graphs and charts. This aspect is particularly advantageous, as effective visualization can sometimes be the key to spotting trends that are not immediately apparent in raw datasets.
However, not all users find the software flexible for advanced analytics. Some data scientists may feel constrained by the tool’s predefined models, which can limit experimentation. Yet, for businesses looking to quickly implement predictive analytics without a steep learning curve, the ease of use can often outweigh such limitations.
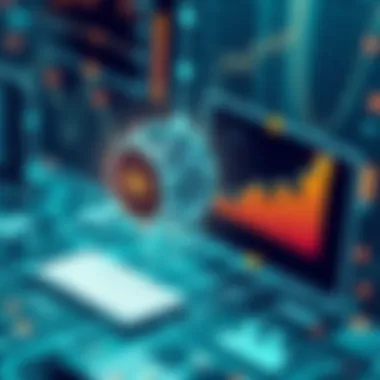
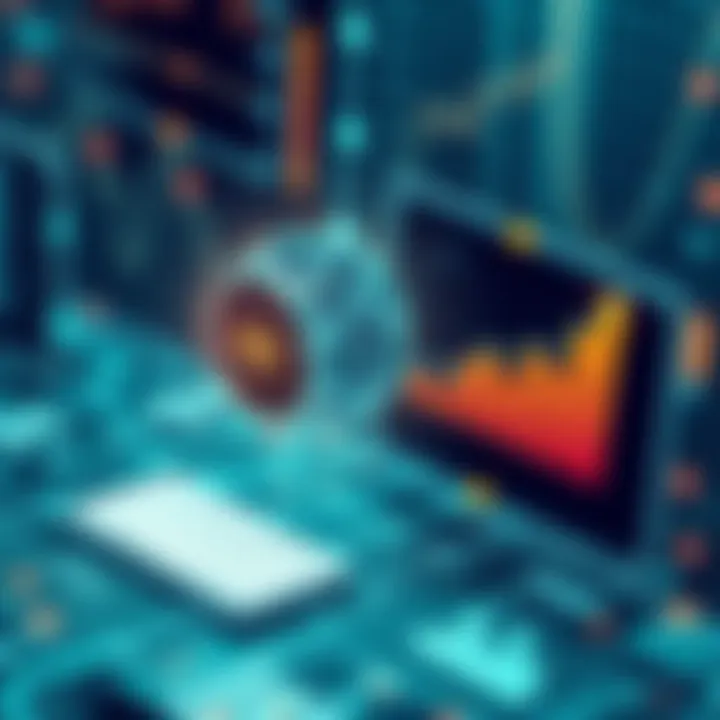
Use Cases
When it comes to practical applications, Software A shines in sectors like retail and finance. Its capabilities in customer segmentation and sales forecasting utilises historical data to predict future patterns. Companies have reported a marked improvement in campaign efficiency due to the insights gained from these analytics. For instance, retailers can optimize inventory levels based on projected demand, thus reducing excess stock while improving customer satisfaction.
Nonetheless, one must consider the scalability of these use cases. While effective for medium-sized businesses, those with global reach might find this software lacking the robust features needed to operate in multiple markets simultaneously.
Client Feedback
Feedback from clients highlights the software’s ability to deliver insights rapidly, which is a great selling point. Many appreciate the customer support they receive, which is credited as being proactive and responsive. Users often mention the appreciable learning materials available, making it easier for new employees to get up to speed with the software.
Yet, some clients express concerns regarding the software's pricing tier. As businesses expand and require more advanced features, the cost can climb rapidly leaves some budget-conscious organizations in a tight spot.
Software B: Overview and Features
Core Functionalities
Software B is known for its advanced algorithms and machine learning capabilities. What sets it apart is its adaptability, as it allows users to train algorithms specific to their business needs. This means that companies in fields such as healthcare or manufacturing may tailor the software to track unique performance metrics that best serve their operations.
A downside, however, is that this adaptability can come with a steep learning curve. Users without a technical background may find themselves overwhelmed by the options for model customization and data input requirements, which can slow down initial implementation.
Use Cases
Software B has carved out a niche in predictive maintenance and demand forecasting. For instance, manufacturers employing this software report a significant drop in equipment failure rates—saving costly downtime. The analytics generated allow for timely interventions in machinery maintenance, which can lead to improved operational efficiency.
However, not all sectors may benefit from such specialized use cases. Smaller businesses might find that with such a bespoke tool, they are paying for features they may never truly exploit.
Client Feedback
Clients generally appreciate the depth of analytics provided by Software B. Many highlight the predictive accuracy as a key advantage, which can lead to better business decisions. Moreover, the community support around this software is noteworthy, with many users sharing tips and tricks online.
On the flip side, some users mention that the interface could be more intuitive. For those who are more visually oriented, navigating the intricacies of the software might feel like searching for a needle in a haystack.
Software C: Overview and Features
Core Functionalities
Software C prides itself on being highly integrative with existing data infrastructure, which is valuable for businesses looking to enhance their analytics capabilities without overhauling their current systems. Its core functionalities include real-time data processing and automated reporting capabilities, which help streamline business operations.
However, it tends to rely heavily on cloud infrastructure. For organizations that are hesitant about data security or dealing with sensitive information, this may raise some red flags.
Use Cases
In the marketing sector, Software C excels in personalized marketing campaigns. By analyzing consumer data, it helps craft targeted ads that resonate with specific demographics, ultimately increasing conversion rates. Many businesses have experienced noticeable improvements in ROI from their marketing efforts, thanks to insights provided by this tool.
Yet, there can be challenges with customization. Companies that require more tailored advertising strategies may find the software’s capabilities somewhat generic, potentially limiting its effectiveness.
Client Feedback
Users frequently commend the software’s ease of integration with other tools. The ability to link seamlessly with various platforms saves time and increases efficiency. The available support resources and ongoing updates contribute to a positive user experience.
Nonetheless, clients have pointed out that the pricing model may not suit all—smaller businesses might struggle to maintain the ongoing costs associated with the software as they grow.
Comparative Analysis of Predictive Analytics Software
The landscape of predictive analytics is vast and continuously evolving. Evaluating these software options side by side is not just beneficial but essential for ensuring that the chosen solution aligns with organizational goals and capabilities. By conducting a comparative analysis, decision-makers can uncover how different software stacks up against each other regarding performance, user experience, cost, and the specific needs they aim to address.
Comparative analysis plays a pivotal role in mitigating the risks associated with large investments in software solutions. It allows professionals to harness data-driven insights, ensuring they make choices based on empirical evidence rather than gut feelings. Such informed decision-making can lead to improved operational efficiencies and enhanced results.
Performance and Accuracy Metrics
When it comes to predictive analytics, performance and accuracy are non-negotiable. Users expect software that can deliver precise predictions based on historical data. This accuracy significantly influences the kind of decisions businesses will make, whether it's optimizing supply chain operations or predicting market trends.
Typically, evaluating these metrics involves looking at several factors:
- Predictive Accuracy: How often the software's predictions come to fruition. Users often rely on statistical measures such as R-squared or Mean Absolute Error to gauge this.
- Speed of Analysis: Many cases require quick turnarounds, and so the ability of software to process data swiftly is crucial.
- Scalability: Can the software maintain its performance as data volumes grow? This often determines how effective the software will be in the long run.
"Dependability reduces risk. When the software you choose is backed by solid performance metrics, you're not just making a choice; you're building confidence in your organization's future."
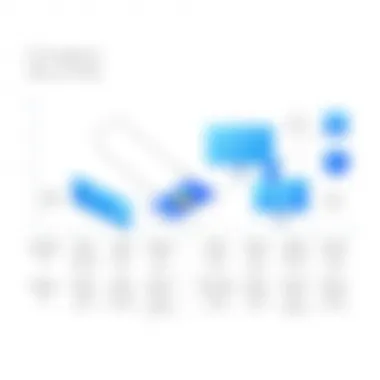
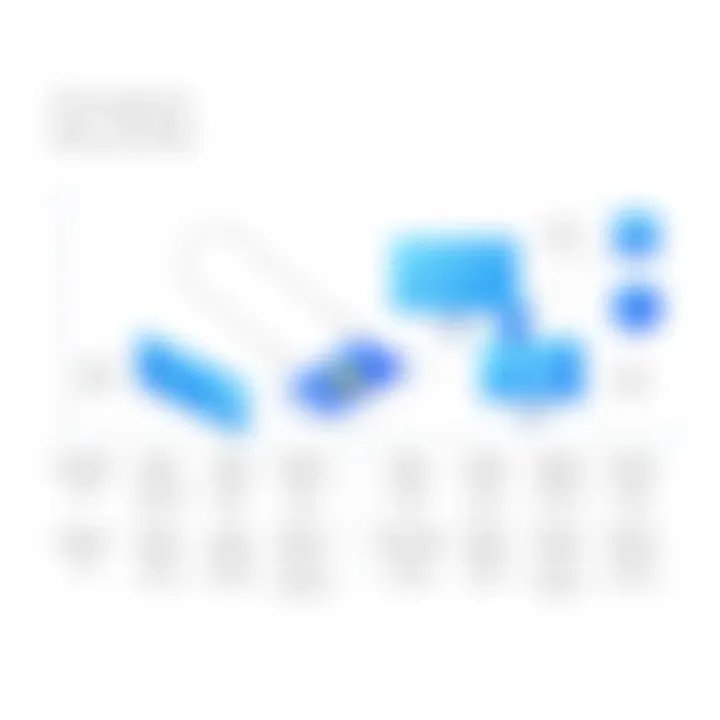
Cost-Benefit Analysis
Cost is naturally a critical factor when choosing predictive analytics software. However, a mere price tag doesn't tell the whole story. Thus, conducting a thorough cost-benefit analysis becomes imperative. This evaluation shouldn't focus solely on the initial investment, but also extend to:
- Total Cost of Ownership (TCO): Consider not just the purchase price but also implementation, training, and ongoing support costs.
- Return on Investment (ROI): A prospective user should weigh the expected gains against the total investment. Tools that can streamline processes or enhance analytics capabilities often result in sizable ROI over time.
- Opportunity Costs: What opportunities might be missed by not investing in a higher-quality software solution? Sometimes, spending a bit more on a reputable software can facilitate climbs in productivity that lower-cost options cannot match.
A well-rounded cost-benefit analysis allows decision-makers to evaluate the tangible and intangible benefits, ensuring they don’t end up with buyer’s remorse down the road.
User Experience and Support
Good software shouldn't just work; it should also feel intuitive to use. A complicated interface can hinder productivity, leading to frustration among users. Thus, examining user experience extends beyond aesthetics—it's about functionality. Factors to consider include:
- Ease of Use: A simple, user-friendly interface helps teams adopt the software faster.
- Customizability: Can the software adapt to the user's specific workflows? Flexibility can significantly boost user satisfaction.
- Support Systems: Tech issues are inevitable, and having responsive support can make a world of difference. The availability of user documentation, community forums, and dedicated support can affect a user's overall experience.
In short, the combination of a pleasant user experience and comprehensive support can dictate how effectively a team integrates the software into their daily operations.
Implementation Considerations for Predictive Analytics
Implementing predictive analytics software is not just a matter of choosing a product and turning it on. It involves a well-thought-out process that ensures the software aligns with your business goals and operational needs. The importance of this section lies in the fact that successful integration can dramatically enhance your return on investment. By understanding the specifics of the implementation process, organizations can better navigate the complexities and extract maximum value from their predictive analytics endeavors.
Initial Setup and Configuration
Getting a predictive analytics solution off the ground starts with its setup and configuration. This initial phase involves a few critical steps:
- System Requirements: Ensure that your hardware and software meet the specifications outlined by the vendor. Not having the right setup can lead to compatibility issues down the line.
- Data Integration: One of the initial tasks must include integrating existing data systems with the new predictive analytics software. This can be a hurdle if older systems are not compatible.
- Customization: Depending on the business, certain features may need customization. For instance, a retail company may require tailored modeling techniques that reflect consumer behaviors, while a financial firm may prioritize risk assessment models.
It's crucial to allow adequate time for this phase, as rushing into user adoption can yield poor results. Proper configuration sets the stage for accurate predictions and meaningful insights down the road.
Employee Training and Resources
The best software in the world will not yield results if employees don’t know how to use it. A solid training program is crucial following the initial setup. Employees must understand the functionalities of the predictive analytics tool to leverage it effectively. Here are some points to consider:
- Hands-On Training: Consider conducting practical sessions where employees can engage directly with the software. This “learn by doing” approach tends to reinforce understanding better than mere theoretical presentations.
- Resource Availability: Provide access to documentation and online resources. In addition to training, having a library of guides and FAQs can empower users to troubleshoot and explore functionalities at their own pace.
- Continuous Learning & Updates: Predictive analytics technology evolves. Ensure that training isn’t a one-off affair but a continuous process. Regular workshops or updates about new features can keep the team up-to-date and engaged.
Monitoring and Maintenance
Even after a smooth implementation, no predictive analytics software can remain effective without ongoing monitoring and maintenance. This stage is often overlooked but can prevent minor issues from snowballing into major setbacks. Key aspects include:
- Regular Performance Checks: Set up mechanisms to routinely evaluate the effectiveness of the predictions being generated. Are they achieving the intended outcomes or falling short?
- Data Hygiene: As new data comes in, it's vital to ensure that the data sets remain clean and updated. Outdated or inaccurate data can lead to misleading predictions.
- Feedback Loops: Establish feedback systems where users can report issues or offer suggestions for improvement. This grassroots input can lead to actionable insights that drive better performance and user acceptance.
In summary, the right implementation strategy can make or break the efficacy of predictive analytics software. By paying thorough attention to the initial setup, investing in employee training, and committing to monitoring and maintenance, companies stand to gain significantly from their predictive analytics investments.
“The journey of a thousand miles begins with a single step.” - Implement wisely, and the rewards that follow can be immense.
Future Trends in Predictive Analytics Software
In the fast-paced world of technology, staying ahead of the curve is not just a strategy; it's a necessity. Predictive analytics software is at the forefront of this evolution, allowing businesses to harness data to anticipate future trends. Understanding the impending shifts in this landscape is crucial for decision-makers, IT professionals, and entrepreneurs looking to leverage these tools for competitive advantage.
Key Elements of Future Trends
The future of predictive analytics is bright, characterized by rapid developments in artificial intelligence, shifts in market demands, and evolving regulatory landscapes. By keeping an eye on these elements, organizations can identify opportunities and risks that arise from these changes.
"Staying informed on predictive analytics trends isn’t just about technology; it’s about ensuring strategic alignment in an ever-changing landscape."
Artificial Intelligence and Machine Learning Integration
The incorporation of artificial intelligence and machine learning into predictive analytics is not simply a passing fad; rather, it represents a fundamental shift in how these systems operate. AI algorithms allow predictive models to learn from historical data and improve over time without human intervention. This leads to:
- Enhanced Predictive Accuracy: By utilizing large datasets, AI can identify patterns that might slip under human radar.
- Automation of Insights: The capability to generate insights automatically saves time and reduces the potential for errors in interpretation.
- Customizable Solutions: Businesses can tailor predictive models to specific objectives, adapting as new data arises.
As AI technology continues to evolve, the integration with predictive analytics tools will likely produce even more sophisticated systems capable of complex predictions, driving more informed decision-making.
Emerging Market Needs
The needs of the market are perpetually transforming. As sectors like healthcare, finance, and retail grapple with challenges arising from a globalized economy, the demand for predictive analytics will surge. Custom analytics solutions focusing on specific industry needs will become paramount. For instance:
- Healthcare: There’s a growing need for analytics that can help track patient outcomes and predict disease outbreaks.
- Retail: As consumers increasingly shift online, inventory and customer behavior analytics will be crucial.
- Finance: Predicting market fluctuations and customer creditworthiness is becoming more complex, requiring advanced analytical tools.
Keeping pace with these trends allows businesses to not just react to changes but also proactively shape their strategies aligning with market needs.
Regulatory Considerations
As predictive analytics software becomes more powerful, regulatory scrutiny is bound to intensify. Compliance with data protection laws such as GDPR and CCPA necessitates a careful approach to data usage. Organizations must consider:
- Data Security: Protecting sensitive information is paramount. This can involve implementing stricter security measures and encryption techniques that ensure data safety.
- Transparency in Algorithms: There may be growing demand for transparency in how predictive models operate, especially when decisions directly affect individuals.
- Ethical Implications: Organizations need to be mindful of ethical considerations, such as biases in data which can lead to unfair outcomes.
As these regulatory dynamics evolve, organizations must remain nimble to adapt their practices to align with the latest policies, ensuring both compliance and integrity in their analytical efforts.