Understanding SPC Data for Quality Control and Improvement
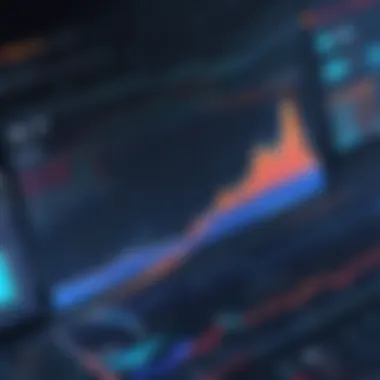
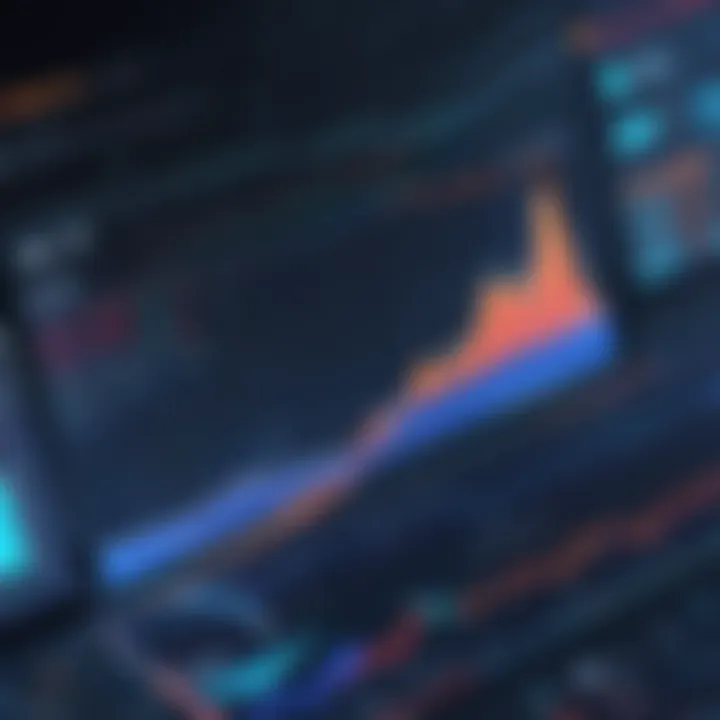
Intro
Statistical Process Control (SPC) is an essential tool in quality management used to monitor and control processes. SPC data is vital for organizations aiming to enhance operational efficiency and maintain product quality. This article aims to provide a detailed exploration of SPC data, its collection methods, applications, and practical implementation strategies. Understanding these elements can significantly influence decision-making within organizations.
Software Category Overview
Definition and Importance
SPC software plays a crucial role in the analysis of data generated during production processes. These tools allow professionals to identify variations in processes, helping to determine whether a process is stable or prone to unexpected changes. By utilizing SPC software, decision-makers can proactively address issues before they escalate, leading to decreased waste and higher quality output.
Key Characteristics
SPC software typically comes with several defining features:
- Real-Time Monitoring: Enables users to observe process data in real time.
- Data Visualization: Offers graphical representations of data, making it easier to interpret.
- Statistical Analysis Tools: Assists in applying statistical methods to assess process performance.
- Alerts and Notifications: Notifies users when processes fall outside predetermined control limits.
These characteristics facilitate a data-driven approach to quality management.
Comparative Analysis of Top Software Options
Feature Comparison
Not all SPC software is created equal. Below is a concise comparison of some popular SPC tools:
- Minitab: Known for its comprehensive statistical analysis capabilities. It offers a user-friendly interface while providing in-depth reporting features.
- SPC for Excel: Integrates SPC charts and calculations directly within Microsoft Excel. Itβs cost-effective and familiar to many users but may lack advanced capabilities of other tools.
- InfinityQS: Focused on manufacturing, this software offers real-time data collection and visualization tailored to various industries.
Pricing Models
Pricing can vary significantly among different SPC software options. Minitab, for instance, operates on a subscription basis, which can be costly for smaller organizations. On the other hand, SPC for Excel offers a one-time purchase model, making it accessible for budget-conscious professionals. InfinityQS tends to offer tiered pricing based on features utilized.
"Choosing the right SPC software depends not only on budget but also on specific organizational needs and size."
By evaluating these aspects, organizations can make informed decisions regarding SPC software that aligns with their operational goals.
Prologue to SPC Data
Statistical Process Control (SPC) data plays a pivotal role in modern quality management. Understanding SPC data provides a framework for analyzing and controlling processes, which is crucial for enhancing efficiency and ensuring product quality. This section outlines the basic concepts and historical significance of SPC, reinforcing its relevance in contemporary industry practices.
Definition of SPC
SPC, or Statistical Process Control, refers to the use of statistical methods to monitor and control a process. This approach aims to ensure that the process operates at its full potential. By employing various statistical tools, like control charts, SPC helps organizations identify variations in processes that may lead to defects or errors. The ultimate goal of SPC is to maintain consistent quality and improve process performance.
Historical context of SPC
The concept of SPC was largely developed during the early 20th century, and its roots can be traced back to the work of Walter A. Shewhart. In the 1920s, Shewhart introduced control charts as a visual tool for analyzing production processes. His pioneering work laid the foundation for modern quality control techniques. In the subsequent decades, SPC gained traction, particularly in manufacturing, where consistent quality was essential for competitive advantage. Today, SPC methodology is applied across many sectors, including healthcare and services, reflecting its versatile nature and impact.
The Importance of SPC Data
Statistical Process Control (SPC) data plays a crucial role in modern industries. Its significance expands far beyond mere metrics. Effective use of SPC data can lead to an enhanced quality of products and services, while also improving operational practices. This section will focus on two key areas of importance: its role in quality management and its impact on operational efficiency.
Role in Quality Management
Understanding how SPC data functions within quality management frameworks is vital. SPC is not just about measuring performance; it is about ensuring consistent quality throughout production processes. By employing control charts and other SPC tools, organizations can monitor processes in real-time. This allows for immediate detection of any deviations from established quality standards.
Key benefits include:
- Predictive analysis: Identifying potential issues before they escalate.
- Root cause analysis: With the data collected, pinpointing sources of variation becomes easier.
- Continuous improvement: Organizations adopt a proactive stance, using data-driven insights to drive quality improvements.
Furthermore, SPC data fosters a culture of accountability. Everyone on the production floor becomes invested in maintaining quality, creating a more engaged workforce. This shift often leads to higher overall morale and productivity, making SPC data an essential element in any quality management system.
Impact on Operational Efficiency
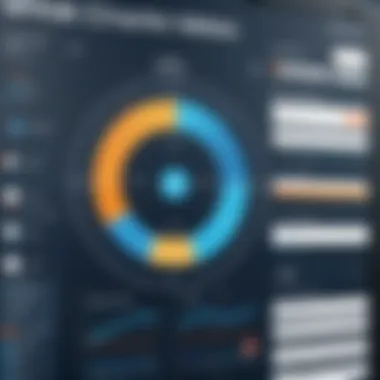
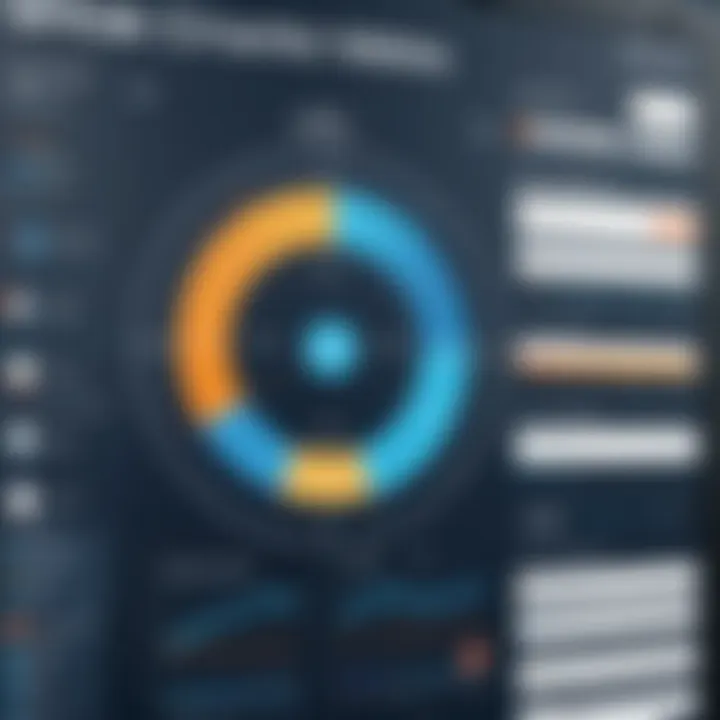
Operational efficiency is another critical aspect enhanced by SPC data. The integration of SPC into daily operations helps organizations streamline processes and eliminate waste. By analyzing trends and variations in data, companies can identify inefficiencies and implement corrective actions.
Some notable impacts include:
- Reduced waste: Understanding process variances leads to more effective resource utilization.
- Improved cycle times: Continuous monitoring helps in shortening production cycles.
- Cost savings: Enhanced efficiency often translates directly into financial savings, benefiting the bottom line.
Key Components of SPC Data
Statistical Process Control (SPC) relies on several key components to effectively monitor and improve processes. Understanding these components is essential for professionals aiming to enhance operational efficiency and ensure product quality. Each component serves a unique purpose in analyzing data, identifying variations, and facilitating informed decision-making.
Control charts
Control charts are a fundamental tool used in SPC. They provide a visual representation of process performance over time. By plotting data points, control charts help in distinguishing between common cause variation and special cause variation. Common cause variation is inherent to the process, while special cause variation indicates an anomaly that requires investigation.
Control charts typically consist of a central line that represents the average of the data and two control limits, which define the acceptable range of variation. If data points fall outside of these control limits, it signals an issue that may need to be addressed. The use of control charts helps organizations maintain consistent quality levels, meet customer expectations, and reduce waste in production.
Process capability analysis
Process capability analysis evaluates the ability of a process to produce output that meets specifications. This analysis measures the relationship between the inherent variability of a process and its specifications. Key metrics involved in this analysis include Cp, Cpk, Pp, and Ppk, which quantify how well a process can deliver consistent results within predetermined limits.
A key benefit of process capability analysis is that it provides insight into how much a process can be improved. It helps identify strengths and weaknesses within the process, allowing for targeted improvements. By understanding process capability, organizations can make strategic decisions regarding process adjustments, leading to enhanced quality and reduced rework costs.
Statistical sampling methods
Statistical sampling methods are essential for collecting data without the need to inspect every single item in a production process. These methods allow organizations to gather representative data that reflects the overall quality of their output. Common sampling methods include random sampling, stratified sampling, and systematic sampling.
Using statistical sampling helps save time and resources while still providing valuable insights into the process. It forms the basis for several other SPC tools, such as control charts and process capability analysis. Organizations adopting these methods can obtain a clearer picture of variability and quality, aiding in more effective decision-making.
"Data-driven decisions with SPC enhance not just quality but overall productivity."
Methods for Collecting SPC Data
The collection of SPC data is fundamental to ensure accurate analysis and quality control in any process. Identifying effective methods for gathering this data plays a pivotal role in the overall success of SPC initiatives. These methods not only streamline data acquisition but also enhance the reliability of information used for decision-making. In addition, precise data collection contributes significantly to minimizing errors and optimizing workflows.
Data sources
Understanding where SPC data originates is crucial for anyone involved in quality management. Data sources can vary widely, depending on the specific applications of SPC. These sources may include:
- Production equipment sensors: These instruments continuously monitor various parameters such as temperature, pressure, and speed.
- Quality inspection results: Data from manual inspections, automated checks, or statistic-based sampling can provide pertinent quality metrics.
- Software systems: Enterprise Resource Planning (ERP) and Manufacturing Execution Systems (MES) provide valuable data regarding production processes and outcomes.
- Customer feedback: Insights from customers regarding product performance can inform quality control efforts.
Ensuring that data sources are reliable and accurate is essential. Inconsistent data can lead to misguided conclusions and ineffective SPC practices. Therefore, decision-makers must establish robust validation processes for each data source.
Data recording techniques
The methods of recording SPC data significantly influence its usability and analytical value. Effective data recording techniques enable professionals to maintain the integrity of the data throughout its lifecycle. Some commonly employed techniques include:
- Manual record-keeping: While this method may seem straightforward, it can introduce human error. However, if managed carefully, it can capture intricate details not easily recorded by other means.
- Automated systems: These systems have advantages such as real-time data capture and less susceptibility to error. Linking automated data collection tools to other software facilitates seamless analysis.
- Mobile applications: Increasingly popular, these apps allow for immediate recording and reporting of data directly from the production floor. This helps in decreasing delays and maintaining up-to-date records.
- Barcode or RFID scanning: This technology enhances speed and accuracy in recording data for inventory and quality checks.
Analyzing SPC Data
Analyzing SPC data is crucial for organizations identified by their commitment to quality and efficiency. This section will examine how systematic analysis of this data enables enhanced decision-making and operational effectiveness. Understanding SPC data helps organizations detect variances in process performance, thereby providing an opportunity to respond proactively. It is about identifying potential problems before they escalate.
Statistical methods used in analysis
Statistical methods form the backbone of SPC data analysis. The following techniques are commonly utilized:
- Descriptive Statistics: Basic measures such as mean, median, and mode provide a summary of data characteristics. They help understand the central tendency and dispersion of the process variables.
- Control Charts: These are graphic representations of process data over time. They help in identifying trends, variability, and potential out-of-control conditions. There are various types of control charts, including X-bar and R charts, each suited for different types of data.
- Process Capability Analysis: This method assesses how well a process performs relative to defined specifications. Key metrics such as Cp and Cpk indicate the consistency and capability of the process.
- Regression Analysis: This statistical technique establishes the relationships between different variables within the process. Understanding these relationships can help in predicting future outcomes.
- Hypothesis Testing: This allows for the testing of assumptions related to process parameters, confirming or rejecting these assumptions based on collected data.
The importance of these statistical methods cannot be overstated. They provide objective and quantifiable metrics that facilitate informed decision-making.
Interpreting control charts

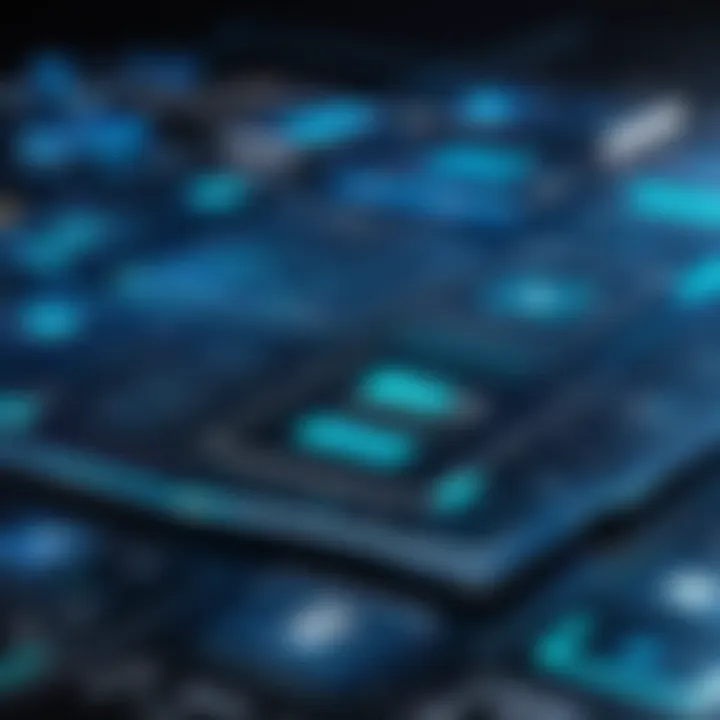
Control charts are essential tools in the SPC arsenal. They allow for continuous monitoring of processes. By interpreting control charts effectively, organizations can maintain product quality and operational integrity.
Key considerations for interpreting control charts include:
- Control Limits: These limits define the acceptable range of variation in a process. When points fall outside these limits, it indicates that the process may be out of control and requires further investigation.
- Trends and Patterns: Monitoring for runs, trends, and shifts in data points can reveal insights about process stability. For example, a series of data points increasing or decreasing consistently suggests a trend that needs attention.
- Variation Types: Distinguishing between common cause variation (natural to the process) and special cause variation (indicative of an issue) is critical. Focus should be on special causes for corrective action.
- Actionable Insights: Determining when to investigate and take action based on the signals from control charts is vital. These signals can range from routine checks to immediate interventions as required.
Effective interpretation of control charts supports proactive measures that maintain quality and enhance productivity.
In summary, analyzing SPC data equips organizations with the necessary insights for process evaluation. Employing robust statistical methods and accurately interpreting control charts leads to informed decision-making and continuous improvement.
Applications of SPC Data
The applications of Statistical Process Control (SPC) data serve as a testament to its pivotal role in enhancing quality and efficiency across diverse sectors. Understanding these applications can elaborate on how SPC data transforms operational outcomes. This section will dissect the significance of SPC data in both the manufacturing and service industries.
Manufacturing Industry
SPC data finds extensive utilization in the manufacturing industry, where precision and consistency are paramount. It aids manufacturers in monitoring production processes to maintain product quality while minimizing waste. Control charts, one of the key tools of SPC, are employed to visualize variations in processes. By analyzing these variations, manufacturers can identify trends and outliers, which prompt necessary interventions to maintain quality standards.
The tangible benefits of implementing SPC in manufacturing include:
- Reduced variation: By consistently monitoring processes, manufacturers can detect deviations early, preventing defects before they occur.
- Cost savings: Better process control leads to decreased scrap rates and rework costs, significantly impacting the bottom line.
- Enhanced forecasting: SPC provides valuable data that can aid in predicting future performance trends, allowing for proactive management.
Moreover, the integration of SPC with advanced data analysis tools can facilitate more intelligent operational decisions. For example, employing predictive analytics in conjunction with SPC can enable manufacturers to foresee potential disruptions in the production line, thereby enhancing responsiveness.
Service Industry
While the manufacturing sector prominently features SPC data applications, its significance in the service industry cannot be understated. This sector experiences challenges such as maintaining service quality amidst high variability in customer interactions. By employing SPC methodologies, service organizations enhance their ability to deliver consistent quality.
In the service industry, SPC data can be applied in various areas, including:
- Customer service: Monitoring metrics like response times and resolution rates assists organizations in identifying inefficiencies and training needs.
- Operations management: Service providers can apply SPC to workflow processes, ensuring services are delivered at optimal speed and quality.
- Quality assurance: By analyzing customer feedback, businesses can pinpoint service failures, leading to targeted improvements.
Some key advantages of using SPC in the service industry include:
- Improved customer satisfaction: Consistent service quality fosters customer trust and loyalty.
- Informed decision-making: Data-driven insights enable managers to make more strategic decisions regarding resource allocation and service improvement.
- Operational efficiency: Streamlined processes through SPC applications lead to better resource management and productivity.
"Implementing SPC in service contexts not only increases operational efficiency but also enhances customer experience through systematic quality monitoring."
In summary, the applications of SPC data across both manufacturing and service industries underscore its effectiveness in driving quality enhancements and operational efficiencies. Understanding these applications can help organizations harness SPC data's full potential to foster continuous improvement and adaptation in a competitive landscape.
Challenges in Implementing SPC Data
Implementing Statistical Process Control (SPC) data in a business environment can be met with several obstacles. Acknowledging and addressing these challenges is crucial for organizations striving for quality improvement and operational efficiency. This section delves into two significant challenges: data integrity issues and resistance to cultural change. Understanding these aspects can facilitate a smoother integration process of SPC data into practice, promoting better decision-making and quality outcomes.
Data integrity issues
Data integrity is essential for effective SPC implementation. The accuracy and consistency of data directly impact the analysis and insights derived from SPC methods. When data is unreliable, it can lead to incorrect conclusions and potentially harmful business decisions.
Several factors contribute to data integrity challenges:
- Inaccurate Data Input: Employees may input data incorrectly due to human error. This can lead to significant discrepancies in outputs.
- Poor system integration: Systems that are not well-integrated may result in data silos. Information might not align properly across different platforms, leading to inconsistencies.
- Lack of standardization: If different departments use varying methods to record data, comparisons may become flawed.
- Inadequate training: Staff who are not properly trained to handle SPC methods may misinterpret or mishandle data, further compromising integrity.
To mitigate these issues, organizations can implement comprehensive training for employees to ensure data is recorded correctly. Adopting standard operating procedures (SOPs) for data collection can also help in maintaining integrity. Regular audits and validations of the data can ensure that it meets necessary standards, ultimately providing a solid foundation for analysis and decision-making.
"Without data integrity, the credibility of SPC initiatives is undermined, leading to misguided actions."
Resistance to cultural change
Cultural resistance is another challenge that organizations face when adopting SPC data practices. Changing the culture in an organization to fully embrace the principles of SPC can be difficult, especially in established environments. Employees may be hesitant to shift from traditional practices to data-driven methods.
Key factors underlining this resistance include:
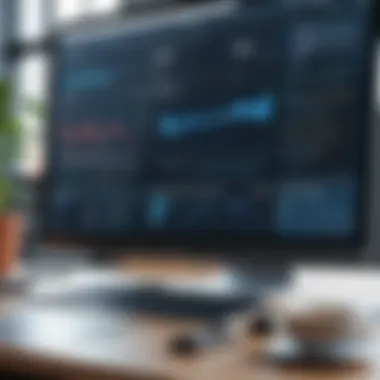
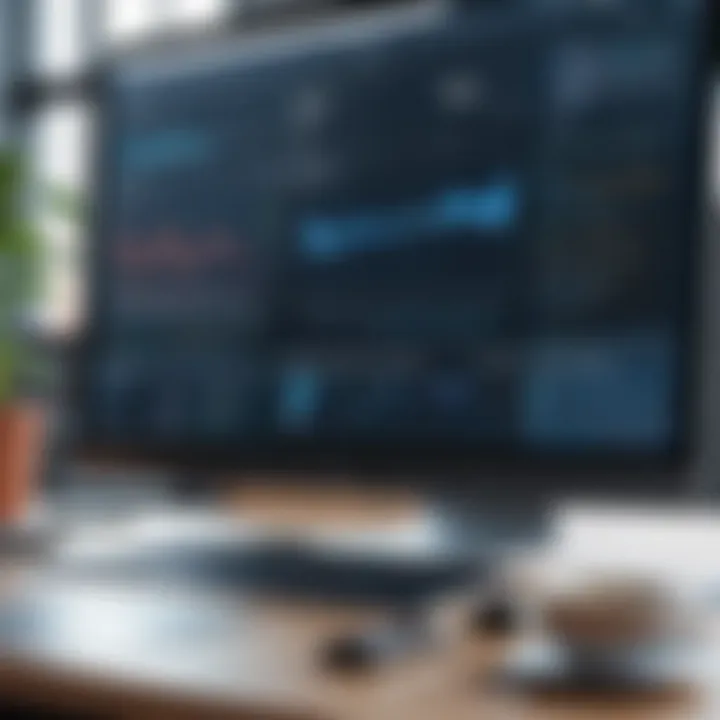
- Fear of change: Employees often feel comfortable with existing processes. Fear of the unknown can result in pushback against new initiatives.
- Misunderstanding SPC's value: If employees do not see the benefits of SPC, they may regard it as a burdensome requirement rather than a useful tool for improvement.
- Leadership support: Without strong commitment from leadership for SPC initiatives, it can be challenging to motivate employees to embrace change.
- Lack of involvement: If employees are not involved in the implementation process, they can feel alienated. Foster engagement by involving them in discussions and decision-making around SPC data usage.
Addressing cultural resistance requires a well-planned change management strategy. Organizations should focus on communicating the benefits of SPC, providing adequate training, and demonstrating organizational commitment to data-driven decision-making. Engaging stakeholders and showing how SPC can lead to improved performance will help foster a culture that embraces quality and continuous improvement.
Best Practices for Effective SPC Implementation
Effective implementation of Statistical Process Control (SPC) data techniques is critical in enhancing quality and efficiency in various processes. The importance of best practices cannot be overstated. Properly executed, these practices lead to significant improvements in decision-making and operational performance. Organizations that adopt these methods not only realize immediate benefits but also establish a foundation for sustainable long-term success.
Training and development
Training and development form the backbone of successful SPC implementation. When team members are well-versed in SPC principles, the accuracy of data interpretation and application improves drastically. Programs should focus on:
- Understanding SPC methodology: Employees need a clear grasp of concepts like control charts, process capability, and variation analysis. Only then can they effectively utilize these tools.
- Hands-on training: Simulations and case studies can provide practical knowledge, enabling workers to apply SPC concepts in real-world scenarios.
- Continuous education: The field of quality control evolves rapidly. Regular workshops and update sessions can keep team members informed about the latest trends and tools in SPC.
Implementing training methods with frequent knowledge checks helps in reinforcing understanding and ensuring retention of key concepts. This investment leads to better data integrity and more effective use of SPC tools, resulting in enhanced quality management.
Continuous improvement initiatives
Implementing continuous improvement initiatives is essential for maintaining momentum in SPC efforts. This involves creating a culture that prioritizes ongoing assessment of processes and outcomes. Strategies include:
- Regular reviews of SPC data: Analyzing trends and patterns provides insights into areas needing improvement. This can lead to timely adjustments in operations, enhancing overall productivity.
- Feedback mechanisms: Open channels for communication encourage employees to share observations and suggestions. This can lead to innovative solutions to persistent issues.
- Setting measurable goals: Organizations should establish clear benchmarks that SPC initiatives aim to achieve. Tracking these objectives helps in measuring success and identifying areas for refinement.
By fostering a mindset of ongoing improvement, organizations not only enhance their current SPC methodologies but also prepare themselves to adapt and evolve as industry needs change.
"Success in SPC implementation originates from building a culture of continuous learning and improvement among team members. The people are the most critical element."
Adopting these best practices lays a strong foundation for SPC data systems. They promote an understanding that extends beyond mere number-crunching to form a central part of the decision-making process. Overall, engaging in training and continuous improvement initiatives significantly amplifies the potential of SPC in enhancing product quality and operational efficiency.
The Future of SPC Data in Industry
As industries evolve, the significance of SPC data continues to grow. Its ability to provide insights into quality control and process efficiency makes it a valuable asset for organizations. The future of SPC data is shaped by several factors that intertwine with technological advancements and changing business needs. Understanding these developments is vital for decision-makers, IT professionals, and entrepreneurs who aim to maintain a competitive edge.
Integration with new technologies
The integration of new technologies into SPC data practices offers transformative benefits. Tools such as Machine Learning, Artificial Intelligence, and Internet of Things (IoT) are now being utilized to enhance data collection and analysis. These technologies enable real-time monitoring, which improves response times to deviations in the production process.
Consider the following benefits of integrating new technology with SPC data:
- Real-time data collection: Traditional methods often involve delays in data recording. Modern sensors and IoT devices can collect data instantly, allowing for immediate action when quality issues arise.
- Predictive analytics: By employing machine learning algorithms, businesses can predict future trends based on historical SPC data. This proactive approach helps in preventing defects before they occur.
- Automation: Automated systems can facilitate seamless data input into SPC frameworks, reducing human error and increasing accuracy.
The adoption of these technologies requires understanding on how to leverage them within existing SPC structures. Fostering a culture of technical adaptability will be paramount in transitioning to these advanced methodologies.
Trends in data analysis
As the volume of data generated increases, so do the trends in data analysis. The future prominent trends include:
- Cloud Computing: Many organizations are shifting SPC data to cloud-based platforms. This allows for easier access and better collaboration among teams, facilitating data sharing and remote monitoring.
- Big Data Analytics: With the capability to analyze large datasets, businesses can extract deeper insights. This includes understanding complex patterns that may not be visible in smaller sets of data.
- Data Visualization Tools: The demand for easy-to-understand data presentations is on the rise. Tools that create intuitive dashboards and visual reports help stakeholders grasp complex data trends without extensive statistical knowledge.
"In an era where data drives decisions, ignoring advancements in data analysis can lead to missed opportunities for improvement."
Maintaining pace with these trends is essential. Organizations that can effectively employ these analytical techniques within their SPC processes will likely improve operational efficiency and product quality.
In summary, the future of SPC data in industry lies in its ability to evolve alongside emerging technologies and analysis methods. By integrating these advancements, organizations can assure quality while also enhancing their bottom line.
Finale
As we reach the end of our exploration of SPC (Statistical Process Control) data, it is essential to recognize its critical role in modern quality management and operational effectiveness. In this article, we have traced the significance of SPC data through its definition, applications, and future prospects. The conclusion serves not only as a summary but also emphasizes the merits of implementing SPC methodologies within organizations.
Understanding SPC data enables organizations to maintain consistent quality standards and improve overall process efficiency. By utilizing statistical techniques, companies can identify variations in production, leading to more informed decision-making. This allows for timely interventions that prevent defects and waste, ultimately safeguarding the bottom line.
The benefits of SPC data are manifold:
- Improved quality outcomes: Organizations that engage with SPC data can significantly reduce defects and enhance customer satisfaction.
- Enhanced operational efficiency: Streamlined processes through the use of control charts and process capability analysis often result in reduced production costs and increased profitability.
- Data-driven culture: Fostering a culture that values data analysis promotes continuous improvement and employee engagement.
Despite its advantages, there are considerations that managers must keep in mind when adopting SPC. These include ensuring data integrity and fostering a workplace environment that is open to the cultural shifts needed for successful implementation.
In summary, SPC data is more than just numbers and graphs; it reflects the heartbeat of an organization's commitment to quality and operational excellence. As industries evolve with technology, consistently refining the strategies surrounding SPC data will be crucial. Vigilant attention to trends in data analysis could shape unprecedented pathways for growth and improvement. As organizations strive for success, embracing SPC principles becomes increasingly relevant, making this exploration a valuable resource for decision-makers and professionals alike.