Workday Forecasting: Methodologies and Best Practices
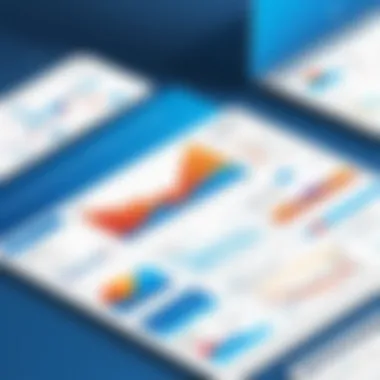
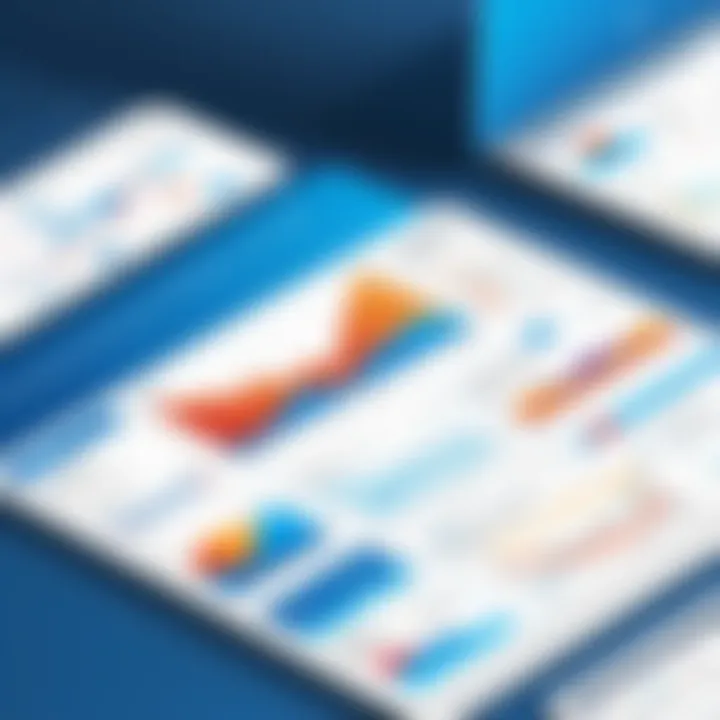
Intro
Workday forecasting is a vital component in the landscape of organizational management. The ability to predict work patterns can drastically affect resource allocation, enhance productivity, and inform strategic planning. By employing various methodologies and best practices, organizations can transform raw data into actionable insights. This article aims to dissect these methodologies, highlight significant data analysis techniques, and unveil best practices essential for effective workforce management.
Software Category Overview
Definition and Importance
Workday forecasting software encompasses tools designed to analyze workforce trends and predict future needs. This technology is significant in the contemporary business environment, where the demand for efficiency and agility is paramount. Organizations that implement robust forecasting methods are better equipped to address unforeseen challenges, allocate resources wisely, and optimize overall operational efficiency.
Key Characteristics
When exploring workday forecasting software, several key characteristics emerge that define their effectiveness:
- Data Integration: Seamless integration with existing HR systems, payroll, and other databases ensures accuracy in predictions.
- User-Friendly Interface: A well-designed interface fosters ease of use among decision-makers and IT professionals.
- Advanced Analytics Capabilities: The ability to perform complex analyses on large data sets is crucial.
- Real-Time Reporting: Providing up-to-date information allows organizations to act promptly on insights.
- Scalability and Customization: Solutions should cater to various organizational sizes and unique industry needs.
The implementation of a forecasting tool not only streamlines internal processes but also enables organizations to maintain a competitive edge in their respective markets.
Comparative Analysis of Top Software Options
Feature Comparison
Different software options come with varied features that cater to specific forecasting needs. Noteworthy software solutions include Workday, SAP SuccessFactors, and Oracle HCM Cloud. Here is a brief comparison of these tools:
- Workday: Known for its intuitive user interface and strong analytics. It offers integrated financial and HR data for comprehensive forecasting.
- SAP SuccessFactors: This software emphasizes talent management alongside workforce analytics. It is ideal for larger organizations with complex needs.
- Oracle HCM Cloud: Offers extensive features related to data security and employee self-service options, making it suitable for businesses prioritizing data protection.
Pricing Models
Pricing models can differ significantly among these software solutions:
- Workday: Generally follows a subscription-based model that varies according to the size of the organization and features selected.
- SAP SuccessFactors: Offers flexible pricing based on the suite of services chosen, which allows for tailored solutions.
- Oracle HCM Cloud: Utilizes a tiered pricing model, making it costly for smaller entities but valuable for larger firms requiring comprehensive solutions.
In the realm of workday forecasting, choosing the right software can markedly influence an organization's ability to harness data effectively. Each tool presents unique advantages, emphasizing the importance of selecting one that aligns closely with organizational goals and structure.
Prelims to Workday Forecasting
Workday forecasting serves as a cornerstone for effective organizational management. This section aims to underscore the integral role of forecasting in aligning resources with strategic goals. Workday forecasting is about making informed predictions regarding the demand for resources, primarily workforce, within a certain timeframe. By accurately forecasting demand, organizations can optimize their operational efficiency and enhance productivity.
Defining Workday Forecasting
Workday forecasting refers to the systematic approach of predicting future staffing needs based on various internal and external factors. This includes understanding patterns related to employee performance, turnover, and even market dynamics. At its core, this practice utilizes historical data to project future requirements. Managing a well-informed workforce is essential for companies that wish to remain competitive. The clarity it brings to resource allocation supports not only immediate operational needs but also long-term strategic goals, ensuring that the right talent is available when needed.
Importance of Effective Forecasting
Effective forecasting in the workplace is not just an added benefit but a necessity. It holds several advantages:
- Resource Optimization: Accurate forecasts enables precise distribution of personnel. This can result in lower labor costs and reduced employee burnout.
- Agility and Adaptability: Organizations can respond quickly to fluctuations in demand. Effective forecasting allows companies to adjust plans and resource allocations proactively.
- Strategic Planning: By knowing future needs, decision-makers can devise strategies that align with broader business objectives. This foresight enhances competitiveness and sustainability.
Effective forecasting isn't merely a predictive tool; it's an essential strategy to navigate the complexities of workforce management.
Building a robust forecasting model requires consideration of various elements, such as data integrity and the applicability of different forecasting methods. Emphasizing its significance reinforces the notion that the organizations that prioritize forecasting are better positioned to adapt and thrive in ever-evolving market landscapes.
Methodologies in Workday Forecasting
Methodologies in workday forecasting form a critical backbone for successful organizational planning. Their significance cannot be overstated as they enable businesses to project workforce needs, budget allocations, and operational strategies. Each methodology offers distinct advantages, targeted applications, and necessary considerations that inform a company's strategic direction. Understanding these methodologies equips decision-makers with the essential tools needed to navigate complex forecasting landscapes.
Qualitative vs. Quantitative Approaches
The debate between qualitative and quantitative approaches in workday forecasting is foundational. Qualitative approaches involve subjective judgment, drawing on expert opinions and historical insights. This might involve focus groups or interviews that elicit perspectives from employees and managers. These insights can reveal trends and gaps not easily captured by numbers.
Quantitative approaches, by contrast, rely on numerical data to drive conclusions. These methods utilize statistical tools and software to analyze past patterns, providing a more structured framework for predictions. While quantitative data may present a clear, data-driven picture, qualitative information can humanize numbers and provide context.
Both methods must not be viewed as mutually exclusive. Rather, they complement each other. Many successful forecasting models integrate both qualitative insights and quantitative data, creating a more robust understanding of workforce dynamics.
Statistical Models
Statistical models are essential for drawing inferences about future workforce requirements based on past data. Different statistical techniques can be applied depending on the specific forecasting need. Common models include time series analysis, regression analysis, and moving averages.
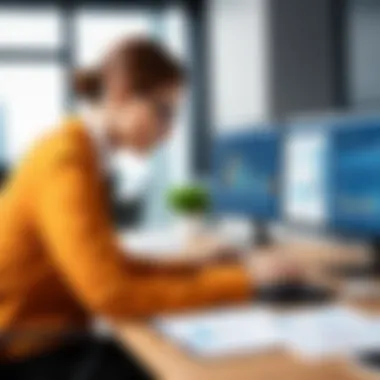
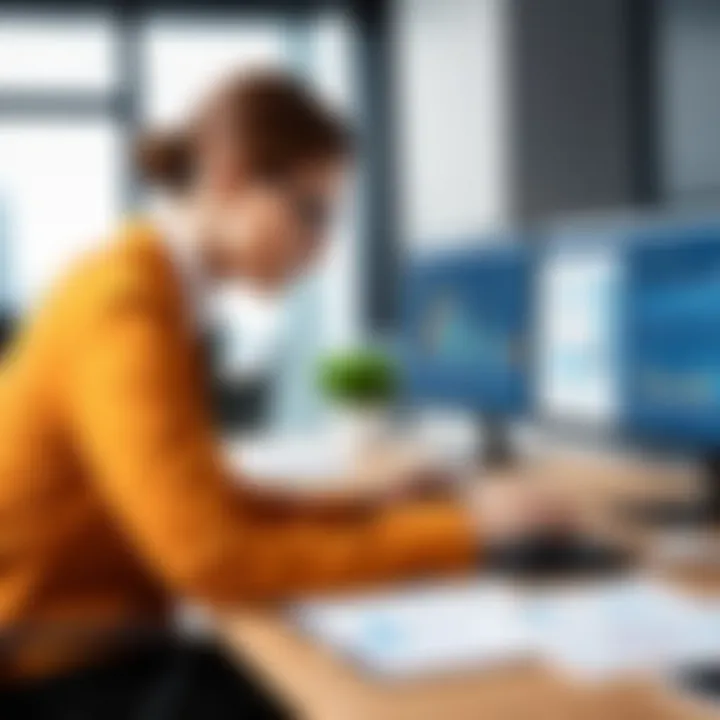
Time series analysis examines data points collected or recorded at specific intervals, helping to identify underlying trends. Regression analysis assesses the relationship between two or more variables, allowing organizations to spot factors that most influence their forecasting accuracy.
Moving averages smooth out fluctuations to identify trends over time. This is particularly useful in volatile environments. Relying on statistical models enhances the precision of forecasts, particularly when supported by good data quality and efforts to minimize biases inherent to human judgment.
Machine Learning Techniques
Machine learning transforms the field of workday forecasting by introducing adaptive methodologies that continuously improve predictions. Unlike traditional statistical models, machine learning algorithms can analyze vast amounts of data from various sources, capturing complexities and patterns often overlooked.
These techniques include decision trees, neural networks, and clustering algorithms. Decision trees visually illustrate choices and their consequences, while neural networks mimic brain function to learn from data inputs. Clustering algorithms classify similar data points, enabling effective grouping of workforce attributes.
"Machine learning enhances the accuracy of forecasts by learning from new data, helping organizations adapt swiftly to changes in conditions."
Incorporating machine learning not only improves accuracy but also reduces time spent on manual data processing. Organizations looking to remain competitive must consider integrating these advanced techniques into their forecasting methodologies. By embracing machine learning, businesses can sharpen their forecasting abilities and respond proactively to evolving demands.
Data Collection for Workday Forecasting
Data collection forms the bedrock of effective workday forecasting. Without accurate and relevant data, predictions can easily go awry, leading to misinformed decisions and resource allocation errors. The process of gathering data involves identifying which metrics and data points are crucial for the specific forecasting goal. These data elements can encompass employee attendance, productivity metrics, historical workload patterns, and external market trends.
The benefit of meticulous data collection cannot be overstated. When organizations invest time in gathering appropriate data, they empower themselves to make informed decisions. Accurate data allows organizations to forecast more reliably, which in turn aids in aligning resources with workload demands. Not only does this enhance productivity, but it also promotes employee well-being by preventing burnout.
Moreover, organizations must consider the sources of their data. Various avenues are available, from internal databases to third-party analytics firms. Each source may present unique strengths and weaknesses. Therefore, it requires a careful assessment to determine the most productive data sources that can deliver actionable insights.
Identifying Relevant Data Sources
Finding relevant data sources is a foundational step in the data collection process. Organizations typically utilize both internal and external data sources. Internal data often includes information from human resources systems, project management tools, or customer relationship management systems. Such platforms provide rich historical data that can serve as a framework for predicting future trends.
On the other hand, external sources can enrich the data pool further. Market research, industry reports, and even social media trends can provide crucial contextual information. Leveraging data from multiple sources ensures a holistic view of the variables that can impact forecasting.
To ensure comprehensive data collection, organizations may consider the following:
- Integrating multiple internal sources for a complete picture
- Leveraging external databases for comparative analysis
- Utilizing public data from government and industry publications
Data Quality and Its Implications
Quality of data is as important as the data itself. Poor data quality can lead to erroneous insights and flawed forecasting. Organizations must establish a set of criteria to assess data quality before using it in analyses. This includes accuracy, consistency, and timeliness. If the data lacks these vital elements, any forecasts developed may lack reliability.
Incorrect or outdated data can skew predictions, leading organizations to make decisions based on false premises. For instance, outdated workforce analytics may suggest a need for additional hires when, in fact, the existing workforce could suffice.
Key implications of data quality include:
- Incorporating regular audits of data sources to maintain accuracy
- Developing standard operating procedures for data entry to ensure consistency
- Training staff on the importance of data integrity and accuracy
Technological Tools for Forecasting
In the realm of workday forecasting, technological tools play a pivotal role in enhancing analytical capability and improving decision-making processes. These tools not only aid in the collection of vast amounts of data but also enhance the analysis and visualization of that data. Therefore, investing in the appropriate forecasting software is crucial for organizations seeking to optimize their operational efficiency. The results from these tools can lead to better resource allocation, increased productivity, and strategic foresight.
Forecasting Software Solutions
Forecasting software solutions are critical for organizations aiming to refine their forecasting processes. These platforms provide robust features that support both qualitative and quantitative analysis. They streamline data collection, enforce data integrity, and facilitate the seamless integration of various data sources. With features like time series analysis, data visualization, and predictive analytics, these tools enable users to derive actionable insights from complex datasets.
When choosing a forecasting software, one must consider several key factors:
- User-Friendliness: The software should be intuitive and easy to navigate. This encourages broader adoption across departments.
- Customizability: The ability to tailor the software to fit specific organizational needs is essential. Every business has unique requirements.
- Scalability: As organizations grow, their forecasting needs change. A good forecasting tool should evolve with these needs.
- Integration Capabilities: Look for systems that can seamlessly connect with existing tools and data sources, improving data flow and enhancing overall efficiency.
Examples of popular forecasting software include Microsoft Power BI, Tableau, and SAP Analytics Cloud. Each of these solutions offers distinct features suited for different organizational strategies.
Integration of AI in Forecasting Tools
The integration of artificial intelligence (AI) in forecasting tools has revolutionized how organizations analyze data. AI-powered tools can detect patterns and trends that may be overlooked by traditional methods. They thrive on vast datasets, allowing businesses to create highly accurate predictions.
Some benefits of incorporating AI into forecasting processes include:
- Enhanced Accuracy: Machine learning algorithms can adapt to new data, enabling more precise predictions over time.
- Automated Insights: AI systems can generate insights without the need for manual analysis, saving time and resources.
- Dynamic Adjustments: AI can automatically adjust forecasts based on real-time data inputs, keeping predictions relevant in rapidly changing environments.
Despite these advantages, organizations must approach AI integration with caution. It is essential to ensure data quality, as AI's effectiveness is contingent on the input data. Moreover, staff may require training to get accustomed to new tools and methodologies. As such, fostering a culture of adaptation and continuous learning within the workforce can enhance the implementation of AI in forecasting tools.
Investing in technological tools for forecasting is no longer an option; it is a necessity for businesses aiming to stay competitive in an increasingly data-driven world.
Challenges in Workday Forecasting
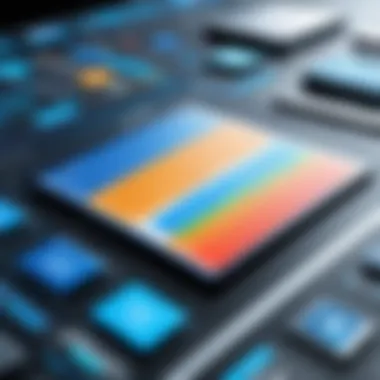
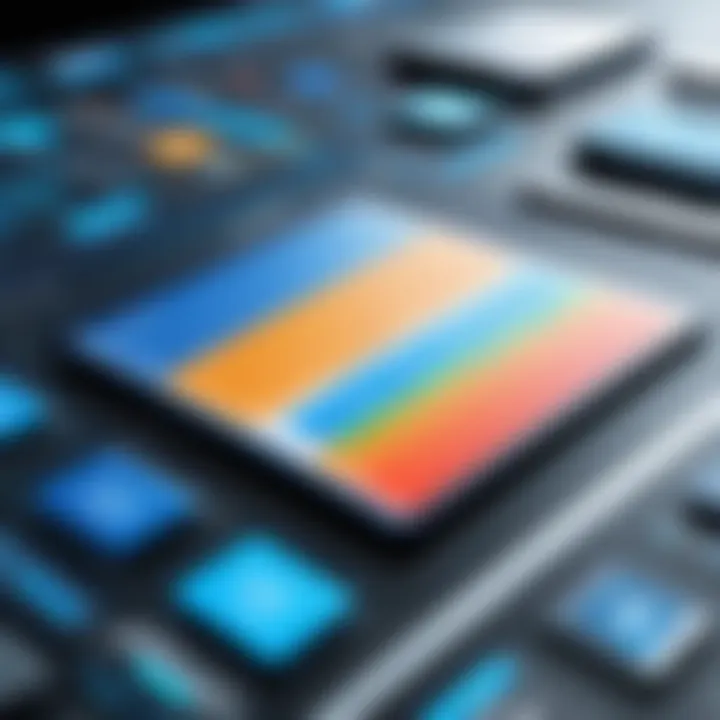
Effective workday forecasting requires navigating several challenges that can impact its utility and accuracy. Understanding these challenges is crucial for organizations that rely on forecasting to make strategic decisions. Each of these elements poses unique concerns that can hinder the forecasting process or lead to suboptimal decision-making.
Data Overload and Analysis Paralysis
One of the most significant challenges faced in workday forecasting is data overload. Organizations often collect vast amounts of data from multiple sources. While having extensive data can be beneficial, it can also lead to analysis paralysis. This is when decision-makers become overwhelmed by the volume of data available, making it difficult to draw actionable insights. To overcome this, it is important to establish a clear framework for data collection and analysis.
Organizations should prioritize data most relevant to their forecasting objectives. Employing effective data management tools can help filter and categorize data, allowing teams to focus on what truly matters. Additionally, creating dashboards that visualize trends may enhance understanding and facilitate quicker decision-making.
Changing Business Environments
Businesses operate in a dynamic environment that is constantly changing. This can include factors like new regulations, shifting consumer preferences, or economic turbulence. Each can substantially alter the forecasting landscape. When organizations fail to adapt their models to these changes, it can result in inaccurate forecasts and misguided strategies. Decisions based on outdated data can affect everything from staffing to budgeting.
To mitigate this risk, organizations must incorporate flexibility into their forecasting methodologies. Employing a continuous improvement model can keep forecasting practices aligned with current conditions. Regularly reviewing and adjusting forecasting parameters ensures that organizations can respond to changes swiftly and accurately.
Seasonality and External Factors
Seasonality and external factors are other significant challenges in workday forecasting. Many businesses experience fluctuations that correspond with seasons or external economic factors. For instance, a retail business may see increased sales during the holiday season, while technology firms might have peaks aligned with new product launches. Ignoring these patterns can lead to miscalculations in workforce allocation or budget planning.
To address this, organizations should include historical data on seasonal trends in their forecasting models. Analyzing past performance during similar periods can provide insights into expected outcomes. Additionally, organizations should stay informed about macroeconomic trends, such as inflation rates and labor market conditions, as these impact forecasting accuracy.
"Understanding and addressing these challenges is fundamental for achieving reliability in workday forecasting."
Navigating the complexities of workday forecasting requires a solid framework to tackle these challenges. Organizations can enhance their decision-making capabilities by developing strategies to address data overload, adapt to changing business environments, and recognize seasonal patterns.
Best Practices for Effective Workday Forecasting
Effective workday forecasting is a cornerstone of organizational success. By implementing best practices, businesses enhance their capability to predict future workforce needs, leading to optimized resource allocation and increased operational efficiency. The elements discussed in this section, such as establishing clear objectives, regularly updating data models, and collaborating across departments, play critical roles in creating a reliable forecasting strategy. Understanding these practices can equip decision-makers with the tools to improve productivity and navigate the uncertainties of business environments.
Establishing Clear Objectives
Setting clear objectives is essential for effective workday forecasting. Objectives provide direction and help to align forecasting efforts with organizational goals. Without well-defined goals, forecasting efforts can become aimless, resulting in inefficient use of resources.
When establishing objectives, consider specific factors like:
- Scope: What aspects of the workforce are being forecasted? Is it staffing levels, skills needed, or project completion times?
- Timeline: Define the period for which forecasts need to be made, whether it is short-term (weeks) or long-term (months or years).
- Metrics: Identify key performance indicators that will measure success. These metrics can include accuracy rates of forecasts, employee satisfaction, or turnover rates.
"Clear objectives provide a roadmap. They help to track progress and adjust strategies accordingly."
Regularly Updating Data Models
Data is dynamic. Thus, regularly updating data models is a practice that cannot be overlooked. As new information emerges or as business environments change, data models need to reflect these realities. By keeping models current, organizations can enhance the accuracy of forecasts. This practice involves:
- Analyzing New Data: Continuously look for relevant data sources and incorporate them into existing models, ensuring that decisions are informed by the latest trends.
- Review Cycles: Establish regular intervals (quarterly or annually) to review models. This helps identify any discrepancies and allows for adjustments based on outcomes from prior forecasts.
- Feedback Loops: Create mechanisms to gather input from users who rely on forecasts. Their insights can help refine models and make them more user-friendly.
Collaborating Across Departments
Collaboration is vital for effective workday forecasting. Different departments possess unique insights that can significantly improve forecasting accuracy. For instance, the sales department might have information about upcoming projects that influence workforce needs, while HR could provide insights about employee turnover trends.
To foster collaboration:
- Interdepartmental Meetings: Schedule regular meetings to discuss forecasting needs and share insights, enhancing understanding and cooperation.
- Shared Platforms: Implement tools that allow for shared access to forecasting data. These platforms ensure that all departments are working from the same data sets.
- Cross-Functional Teams: Create teams with representatives from various departments to work on forecasting initiatives collaboratively. This can enhance overall accuracy and accountability.
Impact of Forecasting on Resource Allocation
The effectiveness of forecasting plays a crucial role in the allocation of resources within an organization. Accurate forecasting allows decision-makers to direct resources more strategically, ensuring that both human capital and financial assets are utilized efficiently. When forecasts are correctly aligned with business objectives, organizations can better respond to market demands, optimize productivity, and enhance overall operational performance.
The insights derived from workday forecasting can significantly inform resource allocation by identifying periods of high demand and successfully aligning workforce distribution accordingly. This leads to several specific benefits:
- Enhanced Productivity: When resources are allocated based on reliable forecasts, teams can work more effectively. Adequate staffing prevents burnout and maintains momentum on crucial projects.
- Reduced Costs: Efficient resource allocation minimizes wastage. By forecasting accurately, organizations can avoid the cost associated with overstaffing or the penalties linked to understaffed departments.
- Improved Flexibility: An organization that forecasts effectively can remain agile. Being able to redirect resources in response to unexpected changes in demand gives a competitive edge.
- Strategic Planning: Good forecasting informs long-term strategies by indicating growth trends and potential operational efficiencies.
"Effective forecasting allows organizations to harness their resources optimally, creating a significant impact on both productivity and cost management."
Organizations must understand that failing to incorporate robust forecasting methods can lead to resource allocation errors, which may detrimentally affect performance and morale. This highlights the importance of aligning forecasting efforts with organizational goals to maximize resource utilization.
Optimizing Workforce Distribution
Optimizing workforce distribution is pivotal for maintaining operational efficiency. It involves the strategic placement of employees to meet forecasted demands effectively. Using data-driven forecasts, organizations can identify when and where their human resources are most needed. Some key focus areas include:
- Skill Matching: Ensuring that the right skills are available in the right departments allows businesses to address client needs promptly.
- Shifts and Schedules: Adjusting shifts based on demand predictions ensures that adequate coverage is maintained without unnecessary labor costs.
- Project Staffing: For short-term projects, forecasting can help determine the best combination of staff to ensure project completion within deadlines.
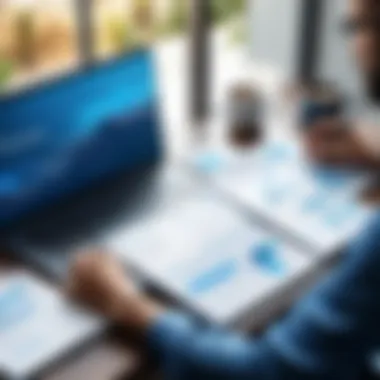
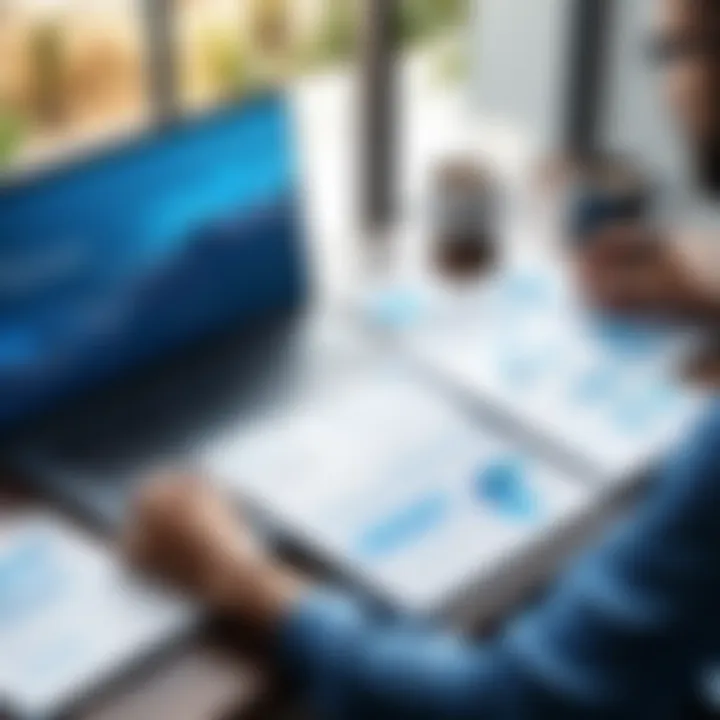
By enhancing workforce distribution, organizations can adapt to fluctuations in demand swiftly while maintaining service quality.
Financial Implications of Accurate Forecasting
Accurate forecasting directly impacts an organization's financial health. It informs budget allocations, helps in revenue predictions, and minimizes financial risks. Predictive analytics allow businesses to create realistic budgets based on expected operational needs. The financial implications include:
- Budget Compliance: Through precise forecasting, organizations can adhere to budgets more reliably, as funds are allocated based on actual needs.
- Revenue Prediction: Accurate forecasts help in anticipating revenue gaps or surpluses, guiding financial strategies and investment decisions.
- Cost Management: Effective forecasting assists in managing costs. By predicting staffing and operational needs, companies can avoid over-spending on unnecessary resources.
In summary, the impact of forecasting on resource allocation cannot be overstated. By optimizing workforce distribution and understanding the financial implications of their forecasting efforts, organizations position themselves for sustainable success and adaptability in an ever-evolving marketplace. This is particularly relevant for decision-makers looking to enhance operational efficiency.
Case Studies in Successful Workday Forecasting
The examination of case studies in successful workday forecasting provides a practical lens through which to view the theories and methodologies discussed in previous sections. Case studies illuminate how different organizations implement forecasting strategies effectively. These real-world examples offer valuable insights into best practices, potential pitfalls, and situational adaptations. With the rapid evolution of business environments, learning from those who have navigated these waters can significantly streamline your own forecasting efforts.
Analyzing successful cases also highlights the tangible benefits of adopting precise forecasting methods. Organizations that utilize effective workday forecasting tend to experience enhanced workforce productivity, improved resource allocation, and more accurate financial planning. Notably, the lessons gleaned from these studies can inform decision-makers when calibrating their forecasting approaches to better align with specific industry needs.
Industry-Specific Examples
Across various industries, the application of workday forecasting has yielded noteworthy results. For instance, in the retail sector, companies like Walmart employ sophisticated forecasting models to predict inventory needs based on historic sales data and seasonal trends. With these models, they achieve a balance between supply and demand, minimizing stockouts and excess inventory, thereby driving profits.
In the healthcare sector, organizations such as the Mayo Clinic have invested in predictive analytics to forecast patient volume and staffing needs accurately. By analyzing historical data and external factors like flu season trends, they can allocate healthcare professionals efficiently, ensuring high levels of patient care.
Additionally, in the tech industry, Google uses advanced algorithms to forecast employee workload based on project timelines and resource requirements. This proactive approach helps maintain efficiency and cohesion within teams, especially during peak periods.
Lessons Learned from Failed Forecasting Efforts
Despite the numerous successes, many organizations have also faced challenging scenarios where forecasting efforts did not yield the expected results. These failures provide critical lessons for those looking to enhance their workday forecasting practices.
For example, a manufacturing company that relied excessively on historical data without considering market fluctuations faced significant overproduction. This situation resulted in increased holding costs and waste. By failing to adapt their models to account for changing market conditions, they limited their operational agility.
Another case involves a financial services firm that implemented a new forecasting tool without adequate training for staff. Misinterpretation of data led to misguided investment strategies, demonstrating the importance of thorough training and user engagement when leveraging new software.
Institutions must remember that workday forecasting is not about merely crunching numbers. It demands a nuanced understanding of business dynamics, technological integration, and staff involvement.
The Future of Workday Forecasting
The importance of understanding the future of workday forecasting can not be overstated. As businesses continue to evolve and adapt to rapid changes, these strategies will be central to effectively managing resources. Significant factors contributing to the upcoming trends include advancements in technology, shifts in workforce demographics, and changes in consumer behavior. Organizations must stay ahead in this dynamic environment to maintain a competitive edge. The capacity to accurately forecast workday requirements ensures not only operational efficiency but also paves the way for strategic planning.
Trends Shaping the Outlook
Several trends are currently influencing the future of workday forecasting. Among these, the following stand out:
- Automation and AI: Artificial intelligence has become crucial in enhancing forecasting accuracy. Tools powered by AI can process vast amounts of data quickly, detecting patterns that human analysts might overlook.
- Remote Work: The rise of remote work has changed how organizations analyze workforce needs. Companies now require flexible models to account for varying workspaces and productivity levels.
- Data-Driven Decision Making: Organizations increasingly rely on data analytics to inform their forecasting methods. This shift demands advanced analytical skills and tools to make sense of large datasets.
These trends are not separate but often interconnected, requiring businesses to approach forecasting with a holistic perspective.
Predictions for Advancements in Methodologies
Looking ahead, several advancements in methodologies are predicted to emerge:
- Enhanced Machine Learning Algorithms: The application of sophisticated machine learning algorithms will refine prediction capabilities. These algorithms will adapt to changing patterns in data, leading to more precise forecasts.
- Integration of Real-Time Data: The integration of real-time data analytics systems will enable organizations to make immediate adjustments to their forecasting models, allowing them to react swiftly to market changes.
- Collaboration Across Departments: Future methodologies will prioritize collaboration by breaking down silos between departments, ensuring that forecasting reflects holistic organizational intelligence.
Organizations that continuously update their forecasting methods and embrace technology will likely outperform their competitors in resource efficiency and decision-making.
Embracing these changes will be essential for companies aiming to thrive in a turbulent business landscape. They must continually evolve their approaches to forecasting, ensuring their methods remain relevant and effective.
End
The conclusion serves as a crucial element in any comprehensive study, including our analysis of workday forecasting. This section synthesizes the key insights gathered throughout the article, providing an overview of the methodologies and best practices that enhance forecasting accuracy.
Summarizing the key insights allows readers to recall essential information efficiently. By distilling the most important points into a concise format, decision-makers can quickly reference strategies and techniques they might implement in their organizations. For instance, recognizing the importance of both qualitative and quantitative approaches in forecasting can guide leaders in selecting the right methodology to fit their unique business contexts.
Moreover, understanding challenges faced in workday forecasting stays essential. Issues such as data overload and changing environments can disrupt even the most well-prepared forecasting efforts. By reflecting on these challenges in the conclusion, practitioners are reminded of the areas needing continual monitoring and adaptation.
Summarizing Key Insights
- Definition and Significance: Workday forecasting aids organizations in anticipating demand for resources, leading to informed decision-making and improved operational efficiency.
- Methodological Approaches: A blend of qualitative and quantitative techniques, reinforced by statistical models and machine learning, offers a robust framework for accurate predictions.
- Data Collection: Identifying and maintaining high-quality data from relevant sources is vital for successful forecasting outcomes.
- Technology Tools: Integrating advanced forecasting software and AI technologies elevates capabilities and precision in predictions.
- Best Practices: Establishing clear forecasting goals, regularly updating data, and fostering collaboration across departments ensures sustained success.
- Challenges Recognized: The impact of external factors and internal data complexities requires constant vigilance and adaptability.
Final Thoughts on Implementation
Implementing effective workday forecasting requires more than just acknowledging its importance. It necessitates a strategic approach, beginning with the clear definition of objectives. Leaders must instill a culture that values data-informed decision-making. Commitment to regular updates in data and models is essential for accuracy in an ever-evolving business landscape.
Furthermore, successful implementation involves breaking down silos within an organization. Collaboration among departments can lead to a more comprehensive view of resources needed, enhancing predictive accuracy. Regular training and investment in technological advancements are also crucial to maintain competitiveness in forecasting methodologies.
In summary, effective workday forecasting hinges on clear structures and an ongoing commitment to adaptation and collaboration. This framing can not only improve operational efficiency but also provide organizations with a competitive edge in resource allocation.