A Comprehensive Examination of Companies Similar to Appen
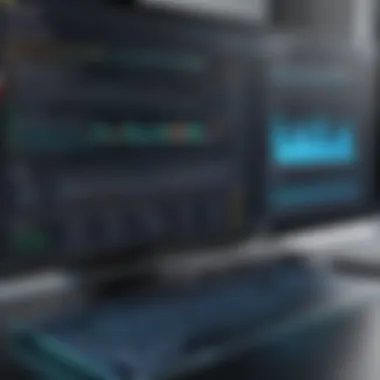
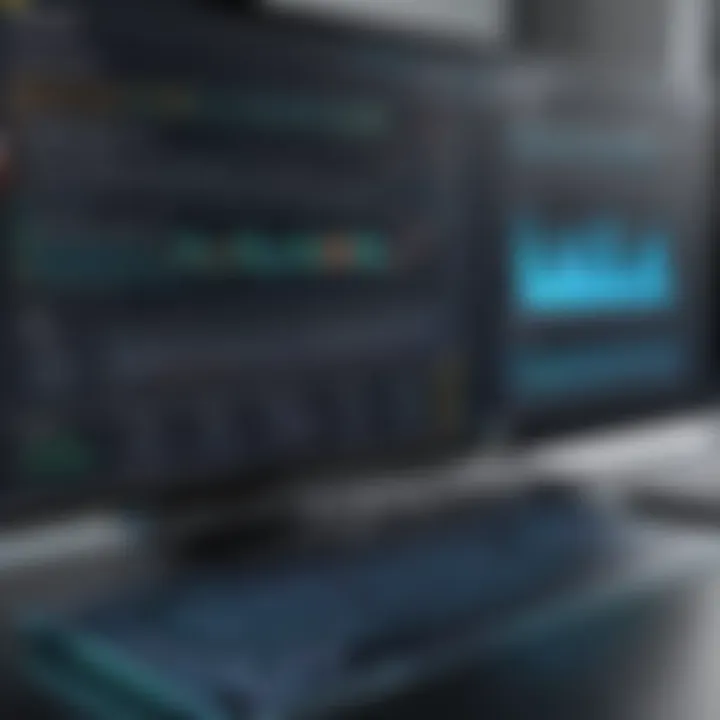
Intro
In today’s data-driven landscape, companies that focus on data annotation and AI training have become increasingly relevant. Appen is recognized as a leader in this domain, offering an array of services that support machine learning and artificial intelligence. However, it is essential to examine the broader spectrum of firms operating in similar capacities. Understanding competitors in this field is not merely an academic exercise. For decision-makers and IT professionals, this knowledge can impact partnership decisions, investment strategies, and overall business outcomes.
Software Category Overview
Definition and Importance
The software category of data annotation and AI training refers to services and tools that facilitate the preparation of data for machine learning models. This process involves classifying, labeling, and organizing data, making it usable for AI algorithms. The importance of this category lies in its role in enhancing the accuracy of AI systems. Quality annotated data is crucial for the success of model training, making this sector pivotal in the AI lifecycle.
Key Characteristics
The firms in this category often share several characteristics that define their operations and offerings:
- Diverse Service Portfolio: These companies typically provide a range of services, from text and image annotation to video analysis and natural language processing.
- Scalability: They design their solutions to handle varying data volumes, which is important for clients with different needs.
- Quality Assurance: Many firms implement rigorous quality assurance processes to ensure high levels of accuracy in data annotation.
- AI Integration: They often leverage their own AI technologies to optimize the annotation process, making it faster and more efficient.
Comparative Analysis of Top Companies
Feature Comparison
To understand the competitive landscape, it is useful to compare prominent players in this space. Below is a brief overview of notable competitors to Appen:
- Lionbridge AI: Offers scalable data annotation services across different formats such as text, audio, and images. They place strong emphasis on quality control.
- Figure Eight: Known for its crowd-sourced platform, Figure Eight excels in providing flexible annotation solutions with an integrated workflow.
- Trianalytic: Specializes in high-quality image and video annotation. They focus on sectors like healthcare and autonomous vehicles, where precision is key.
Pricing Models
Pricing for these services often reflects the complexity and volume of the annotation required. Here are some common models:
- Per Annotation Pricing: Clients are charged based on the number of annotations completed. This model works well for high-volume projects.
- Subscription-Based Pricing: Some companies offer a subscription model that provides access to a suite of tools and services for a monthly or annual fee.
- Custom Quotations: For larger projects or unique service requirements, firms may provide tailored pricing based on specific client needs.
Understanding the pricing structure is essential for effective budgeting and financial planning in data-driven projects.
In summary, companies like Appen are critical in the world of AI training. An analysis of their competitors helps uncover unique attributes and operational frameworks that inform strategic choices in partnerships and investments. This exploration is valuable for those looking to navigate this competitive market effectively.
Understanding Appen's Business Model
Understanding Appen's business model is crucial in evaluating its position and efficiency in the data annotation and AI training industry. Appen has carved out a significant niche by combining solid practices in data collection with innovative technological solutions. This section will explore the fundamental aspects of its operations, mapping out how they function to meet demanding client needs.
Foundational Services of Appen
Appen specializes in various services that are vital for enhancing machine learning models and AI systems. Their core offerings include data annotation, data collection, and linguistic services. Each of these services is designed with precision, ensuring that the data produced meets parameters necessary for high-quality machine learning outcomes.
- Data Annotation: This includes tagging images, transcribing audio, and enhancing unstructured data. The attention to detail is crucial as the quality of annotated data directly influences the performance of AI systems.
- Data Collection: Global reach allows Appen to gather data in different languages and cultural contexts. This offers a diversity of data that is often required for training models.
- Linguistic Services: Appen employs experts who help in localization and translation, crucial for language-specific AI solutions.
These services position Appen as a versatile partner for companies looking to integrate AI solutions effectively.
Target Markets and Clients
Appen serves a diverse clientele ranging from tech giants to startups. Their target markets primarily include software companies, e-commerce, automotive, and healthcare industries. These sectors increasingly rely on data-driven decision-making and, as such, present a considerable demand for high-quality data services.
Some of the notable clients include Microsoft, Google, and Amazon.
- Technology Sector: Heavy user of data for AI enhancement projects.
- Health Sector: Gathering data for predictive analytics.
- E-commerce: Understanding consumer behavior through data.
This diverse market engagement allows Appen to maintain a steady revenue stream while mitigating risks associated with reliance on a single sector.
Appen’s Technological Infrastructure
Technology is the backbone of Appen’s operations. The infrastructure they use is designed to facilitate the seamless collection and annotation of data at scale. Appen utilizes an array of automated tools powered by AI for efficient processes, such as capturing user interactions or extracting relevant data.
"The integration of advanced technology enhances Appen's responsiveness to client demands and contributes prominently to service reliability."
Key Components of Infrastructure:
- Machine Learning Algorithms: These not only enhance data processing but ensure consistency in outputs.
- Cloud-Based Platforms: Allow for real-time collaboration and data sharing among global teams.
- Quality Control Mechanisms: Ensuring that the delivered data adheres to the highest standards.
With such infrastructure, Appen effectively delivers on its promise of providing accurate and significant data to its diverse clientele.
An Overview of Competitors
The competitive landscape in the data annotation and AI training sector is crucial for understanding the market dynamics surrounding companies similar to Appen. Firms in this domain compete for clients seeking effective solutions for their data needs. Recognizing the attributes of these competing entities can provide valuable insights for decision-makers considering partnerships or investments. It enables stakeholders to assess factors like capability, pricing, and service quality, which are pivotal for executing successful projects in artificial intelligence and machine learning.
Key Players in Data Annotation
When exploring the realm of data annotation, several key players come to the forefront. Each firm brings its own strengths, which influence their market positioning. Understanding these players is vital. For instance, Lionbridge and Scale AI have gained recognition for their robust solutions. Lionbridge has a long-standing history with a vast network of data contributors. Conversely, Scale AI leverages advanced technological approaches to drive efficiency.
The adaptability and innovation shown by these companies reflect their potential to meet diverse client needs, an essential factor that businesses analyze when selecting a data annotation provider.
Comparative Analysis of Services
Highlighting Similarities
In analyzing various companies, a notable aspect surfaces in the similarities between services offered by these providers. Many firms, including Appen, Lionbridge, and Scale AI, share a foundation of core services such as text annotation, image tagging, and dataset creation for machine learning models. This commonality highlights their commitment to fostering training datasets that enhance AI capabilities.
The key characteristic of highlighting similarities lies in the reliance on crowdsourced workforces, which ensures a diverse range of data perspectives. This approach has become a beneficial strategy within this industry, allowing companies to manage large volumes of data cost-effectively. Each company's ability to harness these similar service frameworks reflects their broad appeal in the market.
However, a unique feature of this similarity is the variability in quality control processes. Each company applies different standards and protocols, resulting in advantages or disadvantages from a client's perspective. The distinction in quality assurance methods can significantly impact the overall effectiveness of delivered services.
Distinct Service Features
While many companies operate under similar frameworks, distinct service features set them apart. For example, iMerit focuses more on specialized data services tailored for sectors like healthcare or autonomous vehicles. Such specialized offerings appeal to clients requiring niche solutions in particular industries, making them a popular choice among specific client bases.
The key characteristic of these distinct features is their ability to offer tailored services that cater to unique client needs, enhancing customer satisfaction. The unique feature speaks directly to the advantages of having specialized data annotation teams, which can increase project efficiency and quality. However, on the downside, the narrow focus may limit versatility in serving a broader audience.
"In the fast-paced world of AI, the choice of data service provider can profoundly influence project outcomes."
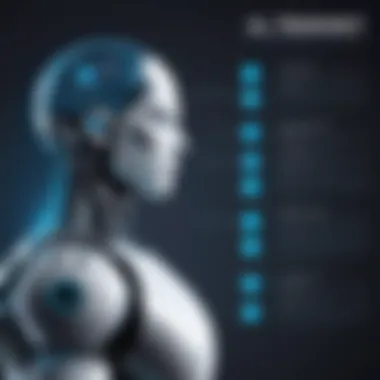
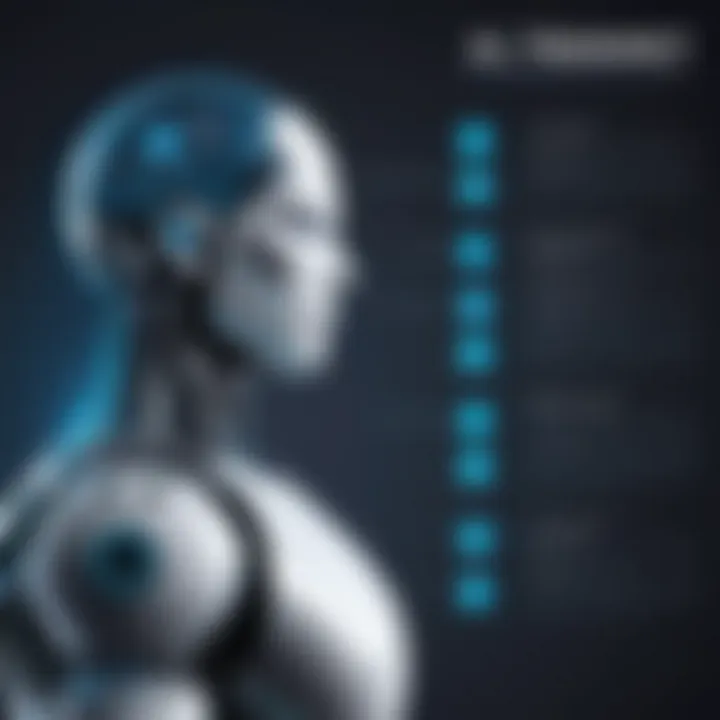
Such knowledge lays the groundwork for strategic partnership considerations.
Lionbridge: A Detailed Exploration
Lionbridge stands as a significant competitor in the domain of data services and AI training, closely resembling Appen in various aspects. The intricate strategies and operational methods of Lionbridge provide valuable insights into how data annotation and linguistic data services can be effectively managed in a competitive landscape. Understanding Lionbridge’s activity helps decision-makers to evaluate it as a potential partner or investment opportunity. As AI and machine learning continue to evolve, analyzing firms like Lionbridge becomes essential for comprehending market dynamics and gaining actionable knowledge about industry standards.
Foreword to Lionbridge
Lionbridge, founded in 1996, is a major player in the field of translation and localization services. With its headquarters in Waltham, Massachusetts, the company operates on a global scale, engaging a vast network of skilled professionals to produce quality outputs. Their vast experience enables them to handle complex projects that not only involve translation but also encompass data annotation, machine learning training, and linguistic validation. This makes Lionbridge a robust option for businesses aiming to integrate diverse data sets within AI systems. The relevance of Lionbridge in today’s data-driven economy cannot be overstated, as companies are increasingly reliant on precise and well-structured data to fuel their AI algorithms.
Service Portfolio
Lionbridge offers a comprehensive service portfolio that covers various facets of data-related needs. The primary services include:
- Data Annotation: This service involves labeling and categorizing data, which is crucial for training machine learning models.
- Content Creation: Tailored content generation ensures that machine learning systems learn from inputs that are not only accurate but also contextually relevant.
- Localization and Translation: Adapting content for different languages and cultural contexts helps businesses reach wider audiences effectively.
- Quality Assurance: Testing and validation ensure that data integrity is maintained throughout the lifecycle.
Lionbridge also offers specialized solutions for specific industries, including automotive, healthcare, and technology. This diversity allows for tailored services that meet unique requirements of various sectors, thus enhancing their market positioning.
Technological Solutions Utilized
Lionbridge utilizes a blend of innovative technologies and platforms to streamline its processes. Their proprietary tools facilitate real-time collaboration and efficient project management, thereby enhancing productivity. Key technologies employed include:
- Artificial Intelligence: AI-driven tools assist in automating parts of the data annotation process, making it faster and more accurate.
- Cloud Platforms: These allow for the secure storage and management of vast amounts of data, ensuring that it is readily accessible to teams across different locations.
- Machine Learning: Through advanced ML algorithms, Lionbridge improves the learning capabilities of its services, enabling continuous enhancement of data quality and relevance.
Lionbridge remains committed to leveraging technology for better service delivery, making it a contender worth examining for those interested in the data services domain. As the demand for data annotation and AI training rises, their methods and tools place them in an advantageous position to capitalize on emerging market trends.
"Understanding the infrastructure and methodologies Lionsbridge applies can provide critical competitive insights for any company involved in AI and data services."
By understanding Lionbridge's offerings and technological foundation, one can appreciate their role in the fast-evolving landscape of AI and data services. This strengthens the dialogue around strategic decisions in partnerships or investments.
Scale AI: Innovations and Services
Scale AI stands as a pivotal player in the realm of data annotation and AI training services, offering distinctive approaches and solutions that cater to an evolving market. Understanding Scale AI’s dynamics is essential for decision-makers seeking to enhance their data handling capabilities and integrate robust AI strategies. This section focuses on the company's profile, unique offerings, and competitive advantages it possesses in technology and services.
Profile of Scale AI
Scale AI, founded in 2016, focuses on providing high-quality training data for machine learning purpose. The company thrives on its strong emphasis on scalability and efficiency, providing businesses with the capacity to manage extensive datasets with ease. Its notable client base includes well-known tech giants, indicating a robust reputation within the data annotation space.
Unique Offerings in Data Services
Scale AI's services stand out due to their versatility and adaptability. The major offerings include:
- Data Annotation: Scale AI provides custom data labeling solutions designed for computer vision, natural language processing, and more. This flexibility allows clients in various industries, such as automotive and healthcare, to have tailor-made solutions.
- Quality Control: Their continuous feedback loop ensures that the data remains consistent and accurate, meeting pre-defined quality benchmarks.
- Rapid Scalability: With a diverse workforce and a strong technological foundation, Scale AI can quickly scale operations, accommodating projects of varying size and complexity.
These unique features enable companies to fine-tune their machine learning models effectively, leading to improved outcomes across different domains.
Technological Competitive Edge
Scale AI harnesses advanced technologies to maintain a competitive edge in the data services sector.
- AI-Enhanced Annotation: The company employs AI algorithms to streamline the annotation process, enhancing speed while sustaining accuracy. This technology reduces human error and accelerates project turnaround times.
- Robust Platform: Scale AI’s platform facilitates easy integration with clients’ existing systems. Its user-friendly interface promotes a seamless workflow, allowing customers to focus on strategic decisions rather than technical hurdles.
- Data Security: With increasing concerns surrounding data privacy, Scale AI prioritizes security measures. Using state-of-the-art encryption and access controls, the company ensures that sensitive information remains protected.
"Scale AI combines human intelligence with artificial intelligence to gain remarkable results in data quality and delivery speed."
Mighty AI: Operational Framework
Mighty AI stands as a significant player in the data annotation space, with its operational framework intricately designed to harness the potential of artificial intelligence and machine learning. Understanding this framework is crucial for stakeholders looking to partner or invest in data service companies. It offers insights into how effective data training can feed into AI models, ultimately influencing decision-making processes in technology and business.
Understanding Mighty AI
Mighty AI operates with a focus on creating high-quality training datasets essential for AI algorithms. Their emphasis is on providing datasets that have undergone meticulous human annotation. This helps in creating reliable machine learning models. One notable aspect of Mighty AI’s operation is its emphasis on community-driven tasks, where skilled annotators contribute to dataset creation. This community-centric approach ensures diverse perspectives and high-quality results.
Furthermore, the company utilizes various tools and technologies that facilitate real-time collaboration and feedback among annotators and project managers. This structure not only promotes efficiency but also enhances the training quality of AI models, making them more accurate and functional in real-world applications.
Data Training Methodologies
Mighty AI employs several data training methodologies that cater to different project requirements. Their approach often incorporates the following techniques:
- Human Annotation: Skilled annotators perform manual tagging and labeling of data, ensuring precision.
- Image Classification: This involves sorting images into categories, necessary for training computer vision models.
- Natural Language Processing (NLP): Annotation of textual data to assist in developing chatbots and language models.
- Active Learning: Data labeling is continuously improved through machine learning feedback loops, refining both the model and the dataset over time.
"The success of AI models heavily relies on the quality of data they are trained on, making the role of companies like Mighty AI indispensable."
These methodologies enable Mighty AI to produce training data that meets the specific needs of various industries, such as automotive, healthcare, and e-commerce. Their operational flexibility and focus on quality result in a robust framework that supports AI advancements, and in turn, delivers significant value to their clients.
Amazon Mechanical Turk: A Crowdsourcing Solution
Amazon Mechanical Turk (MTurk) serves as a significant player in the landscape of crowdsourcing solutions. With its core offering centered around human intelligence tasks, it offers a platform where businesses can leverage the cognitive abilities of a vast crowd of workers. This is important within the context of data annotation and AI training because it provides cost-effective options for companies looking to gather large amounts of data quickly. By tapping into this resource, organizations can significantly enhance their data training processes while maintaining control over quality and scalability.
Profiling Amazon Mechanical Turk
Amazon Mechanical Turk was established by Amazon in 2005. It functions as a marketplace for work that requires human intelligence. Clients, often referred to as "requesters," post tasks that can be completed by workers known as "Turkers." These tasks vary widely, from simple data entry to complex decision-making challenges.
MTurk has gained traction primarily due to its ease of use and accessibility. Requesters can create an account and submit tasks within minutes. Meanwhile, Turkers can select tasks that fit their skills and availability, making it a flexible option for freelancers worldwide. The platform has revolutionized how businesses access human input, optimizing workflows and speeding up project timelines.
Crowdsourcing and Its Application
Crowdsourcing, as facilitated by Amazon Mechanical Turk, involves obtaining information or services from a large group of people rather than traditional employees or suppliers. This method is particularly useful in the context of data services for several reasons:
- Cost Efficiency: Crowdsourcing can significantly reduce labor costs. Many tasks on MTurk can be completed for a fraction of what it would cost with full-time employees.
- Scalability and Speed: When time is of the essence, MTurk offers a rapid solution. Requesters can scale their projects up or down easily, based on requirements.
- Diverse Skill Set: The platform provides access to a wide range of skills. Requesters can choose workers based on specific qualifications, allowing for tailored task completion.
However, there are considerations to keep in mind:
"Quality control can be a challenge when relying on crowdsourced workers. Implementing screening and validation mechanisms is crucial for maintaining the integrity of the data collected."
In practice, MTurk can be applied to various tasks like data annotation, sentiment analysis, and even usability testing. Integrating MTurk into project workflows allows companies to leverage the power of a large workforce effectively. This not only enhances the volume of work accomplished but also enriches the data quality that is vital for machine learning algorithms.
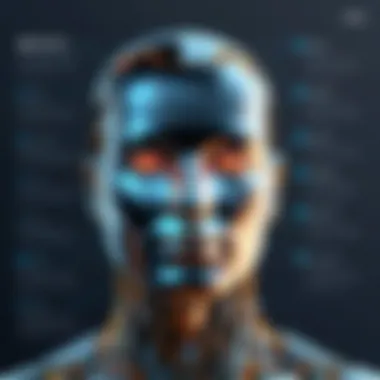
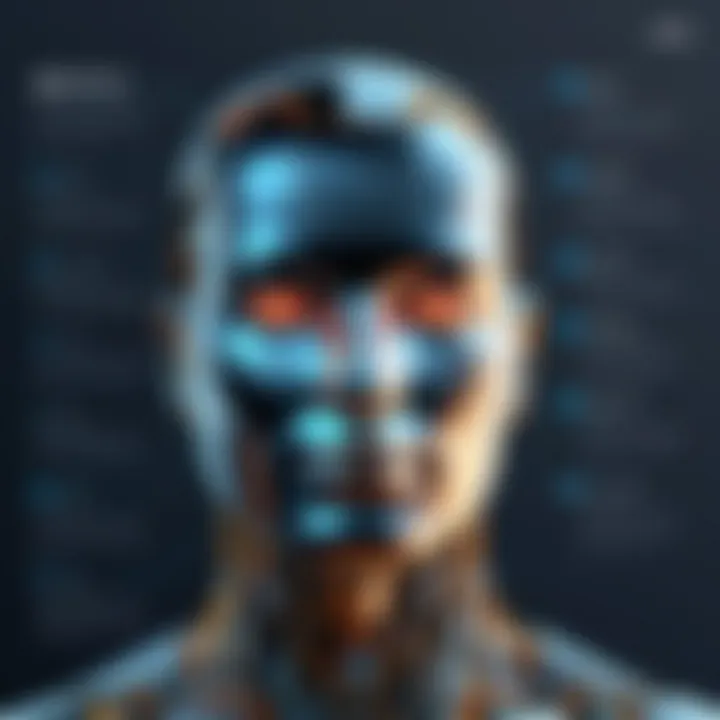
iMerit: Specialized Data Services
The examination of companies similar to Appen necessitates a deep dive into iMerit, a firm that stands out for its specialized data services. iMerit offers unique approaches to data annotation, particularly tailored for various industries. This specialization allows it to meet the advanced data needs of its clients effectively. Understanding iMerit’s operations provides a significant insight into the competitive landscape of data services, especially within data annotation and training.
Prologue to iMerit
iMerit was founded in 2012 and has since developed a reputation for delivering high-quality data services. It emphasizes the use of skilled human intelligence combined with advanced technology. This blend ensures that the data produced is accurate and relevant to its client's needs. iMerit supports a range of domains, including healthcare, e-commerce, and autonomous vehicles. The company employs a large workforce to address diverse data challenges, relying on their expertise to enhance project outcomes.
Key aspects of iMerit include:
- Quality Assurance: Stringent checks are in place to ensure the data meets the required standards.
- Scalable Solutions: The ability to quickly scale operations according to project demands is a critical feature of their service.
- Domain Expertise: Specialists in various fields lend their knowledge to tailor solutions for specific markets.
Industry Applications
The applications of iMerit's services are wide-ranging, affecting several industries significantly. Some areas where iMerit has made substantial contributions include:
- Healthcare: They provide data services that support medical imaging, patient data processing, and clinical data management. Their work helps improve diagnostic tools and patient care.
- E-commerce: iMerit aids in cataloging products, enhancing searchability, and improving user experience on e-commerce platforms. This helps businesses drive sales through better data management.
- Automotive: In the realm of autonomous vehicles, iMerit contributes to the training of AI models with annotated data, enabling machines to interpret real-world scenarios more effectively.
Understanding the breadth of iMerit’s applications is crucial for decision-makers examining partnerships in data services. The focus on industry-specific solutions highlights their adaptability and commitment to meeting client needs. This specialization can be a distinct advantage for companies needing tailored data inputs for machine learning and AI development.
"iMerit's work is vital for industries that depend on precise and consistent data, allowing them to innovate and serve better their customers."
With the knowledge of iMerit's strong position in specializd data services, stakeholders can better evaluate their options within the competitive landscape of data annotation and AI training.
Data Annotation Tools and Technologies
Data annotation tools and technologies play a crucial role in the landscape of data services, especially for companies like Appen. These tools facilitate the process of labeling datasets, a fundamental step in training machine learning models. Each tool comes with its specific features, functionalities, and advantages that can significantly influence the efficiency and accuracy of data annotation projects.
Overview of Tools
In the market, there are various data annotation tools, each designed to address different needs and applications. Some of the prominent tools include:
- Labelbox: Known for its intuitive interface and powerful collaboration features, Labelbox allows teams to work together seamlessly on labeling tasks.
- Amazon SageMaker Ground Truth: This tool integrates machine learning with human annotators for labeling, which can reduce costs and improve efficiency.
- SuperAnnotate: Offers a comprehensive platform that supports various annotation types, including image, video, and 3D data. Its strong focus on user experience sets it apart.
- Prodigy: Designed for rapid data annotation, Prodigy utilizes active learning to prioritize which data should be annotated first, making it suitable for agile projects.
Each of these tools has specific strengths and weaknesses. The choice of tool can depend on several factors like the type of data, project scale, and specific annotation requirements.
Evaluating Effectiveness of Tools
Evaluating the effectiveness of data annotation tools requires a structured approach. Key considerations include:
- Accuracy of Annotations: The primary goal is to produce high-quality labeled data. Tools that incorporate automated quality checks often perform better.
- Ease of Use: The user interface should be straightforward, enabling annotators to focus on the task rather than struggling with the tool itself.
- Integration Capabilities: Effective tools should seamlessly integrate with existing workflows and data systems.
- Cost Efficiency: Budget constraints are a reality. Compare the pricing models in relation to the features offered.
- Support and Documentation: Reliable customer support and comprehensive documentation can significantly impact the user experience and troubleshooting needs.
"The effectiveness of data annotation tools is not just in their features but also how they fit into the workflow of the organization."
Understanding these dimensions helps in selecting the right tools for achieving optimal results in data projects. The ongoing evolution of technologies ensures that continuous evaluation and adaptation are necessary for maintaining a competitive edge.
Industry Trends Impacting Companies
Understanding the industry trends impacting companies in the domain of data annotation and AI training is crucial for stakeholders. Observing the shifts in this landscape helps in recognizing not only the operational challenges but also the growth opportunities that these trends present. Key trends can shape business strategies, influence consumer behavior, and dictate competitive dynamics. Therefore, it is imperative for decision-makers to stay informed about these trends to make astute choices regarding partnerships and investments.
Shift Towards Automation
The shift towards automation profoundly impacts the industry of data services. Automation minimizes human error while increasing efficiency and scalability. Tasks that previously required extensive human labor can now be performed by algorithms and software systems. This transition not only enhances productivity but also reduces operational costs significantly.
- Efficiency Gains: Automating workflows allows for quicker turnaround times in data processing.
- Cost Reduction: Less reliance on manual labor can lead to significant cost savings.
- Scalability: Companies can scale operations rapidly to meet market demands without corresponding increases in workforce size.
However, it’s worth noting that not all tasks can be automated completely. Complex data tasks still benefit from human oversight to ensure accuracy and relevance. Thus, a balanced approach that combines both human intelligence and automation is recommended.
The Role of Machine Learning
Machine learning is another pivotal trend influencing companies in the data annotation sector. This technology specializes in algorithms that enable systems to learn from and adapt to new information. Its role is multifaceted and enhances various aspects of data services.
- Improved Data Quality: Machine learning algorithms can identify patterns and anomalies in data, enhancing the quality of training datasets.
- Personalization of Services: Companies use machine learning to provide customized solutions tailored to specific client needs, thus improving client satisfaction and loyalty.
- Predictive Analytics: Organizations can leverage machine learning for predictive modeling, allowing for better forecasting and strategic planning.
Overall, the integration of machine learning into data annotation services signifies a shift towards smarter, more efficient operations. The combination of these trends not only enhances the capabilities of companies but also positions them strategically within a competitive market.
Ethical Considerations in Data Services
Ethical considerations play a crucial role in guiding the practices of companies involved in data annotation and AI training services. With the growth of artificial intelligence and machine learning, ethical dilemmas have become more pronounced. These challenges, particularly relating to data privacy and worker rights, must be addressed to maintain trust and compliance in the industry. The proper handling of data and fair treatment of labor are not only legal requirements but also essential for sustainable business practices.
Data Privacy and Security
Data privacy and security are perhaps the most critical aspects of ethical considerations in this sector. As companies like Appen operate in environments where sensitive information is prevalent, they face stringent regulations such as the General Data Protection Regulation (GDPR) in the European Union.
Organizations must ensure that data collected is done so transparently and with informed consent. Effective measures need to be in place to protect this data from breaches. Secure storage solutions, encryption methods, and regular audits can bolster security.
Additionally, companies must remain vigilant against potential misuse of collected data. They must implement policies that delineate data usage clearly, allowing customers to know how their information is protected and employed. Sustainable practices in data handling enhance customer confidence and loyalty, which are invaluable assets in the competitive landscape of data services.
"Data privacy is not just a compliance issue. It is a fundamental right that must be respected and protected."
Fair Labor Practices
Equally significant are fair labor practices within data services. As companies deploy extensive human resources for data annotation, the ethical treatment of these individuals must not be overlooked. Often, workers are located in different parts of the world, sometimes in developing economies. Ensuring fair pay, safe working conditions, and reasonable hours helps combat exploitation and builds a reputable brand image.
Implementing clear, fair contracts and providing adequate training ensures that workers are aware of their rights and responsibilities. Moreover, companies can benefit from establishing direct communication with their workforce to address grievances promptly and effectively.
When companies prioritize fair labor practices, they not only contribute to a more equitable workplace but also attract high-quality talent. Workers tend to perform better and remain more engaged when they feel valued and safe.
User Experience and User Interface in Data Platforms
In the realm of data annotation and AI training services, the significance of user experience (UX) and user interface (UI) cannot be overstated. These elements are crucial in ensuring that users can execute tasks efficiently, reducing frustration and enhancing productivity. A well-designed platform fosters user satisfaction and encourages continued use of the services provided, making it an essential focus for companies competing with Appen.
The design of the user interface directly affects how easily users can navigate the complexities of data annotation tasks. Developers must prioritize responsiveness, clarity, and intuitiveness in their designs. Particularly, as these platforms often involve intricate workflows, any complexity in UI can lead to decreases in user confidence and effectiveness.
This importance is amplified by the rapidly evolving landscape in AI technology. As more sophisticated tools become available, companies must meet rising user expectations regarding usability. Ensuring a positive UX/UI experience can serve as a distinctive competitive advantage.
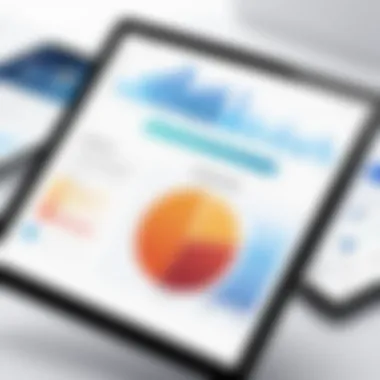
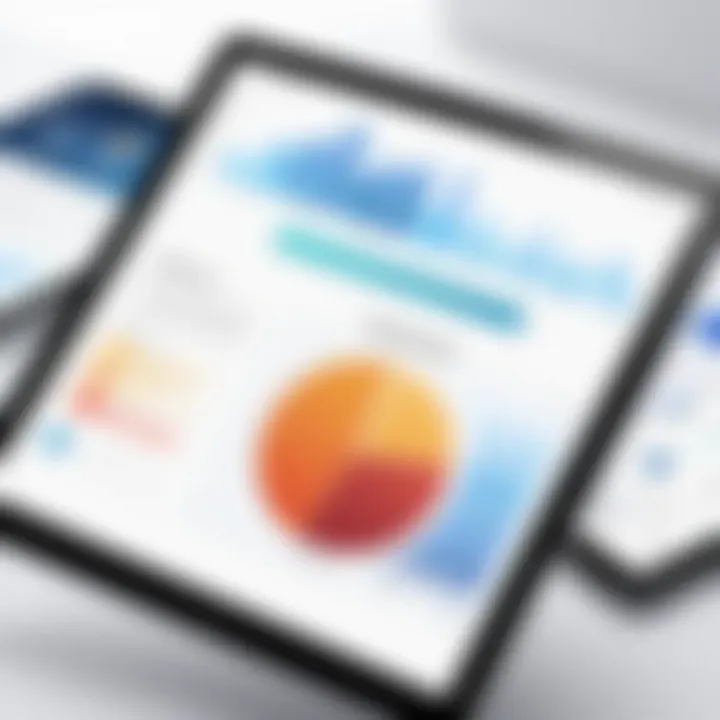
Importance of UX/UI
- Enhanced User Engagement: UX/UI design plays a pivotal role in keeping users engaged. If a platform is visually appealing and easy to use, users are more likely to return to it. This dependency is vital in industries where consistent usage can translate to improved accuracy in data annotation.
- Operational Efficiency: Intuitive user interfaces streamline workflows. Users can accomplish tasks faster when they do not struggle to find necessary features or understand workload management. Improved efficiency correlates with better overall productivity, which is critical for any data services firm.
- Lower Training Costs: A robust UX/UI requires less training for new users. When interfaces are user-friendly, new team members can get up to speed more quickly, thereby minimizing onboarding costs. This efficiency also leads to a shorter learning curve, benefitting operational timelines.
- Feedback and Improvement: A well-executed UX strategy encourages user feedback. Data service firms can utilize this feedback to improve functionality and address pain points within the platform. Thus, responsiveness to user needs not only elevates satisfaction but can drive product evolution.
Best Practices to Enhance UX/UI
Implementing effective user experience and user interface strategies lies within the following best practices:
- User-Centric Design: Always prioritize the end-user when designing interfaces. Gathering direct input from users ensures the platform meets their needs and expectations. User personas can be used to tailor the experience appropriately.
- Consistent User Interface Elements: Consistency across buttons, colors, and layout enhances familiarity. Users build their confidence navigating through a platform when they recognize these elements, making them feel comfortable and efficient.
- Responsive Design: Modern data platforms should adapt seamlessly across various devices and screen sizes. Users expect to have an optimal experience whether they access the platform via desktop systems or mobile devices.
- Frequent Usability Testing: Conduct usability tests regularly. This practice identifies pain points and areas for enhancement. Involving actual users during the testing phase ensures that the feedback is practical and applicable.
"In the tech sphere, where user retention is paramount, investing in UX/UI design can differentiate a company from its competitors."
- Effective Training and Onboarding: Implement comprehensive training materials and tools that guide users through their initial interactions with the platform. Clear documentation and tutorial videos can enhance understanding and operational competence.
By focusing on user experience and interface design, companies in the data service industry can create more engaging, efficient platforms. Such efforts can lead to stronger market positions and more satisfied clients.
Strategic Positioning of Data Service Companies
Strategic positioning is a crucial factor for data service companies. It influences how these companies are perceived in the market and ultimately affects their competitive advantage and sustainability. This section explores the significance of strategic positioning and its various facets.
Positioning involves defining a company's unique place in the market relative to its competitors. For data service companies, this could relate to their specific competencies in data annotation, machine learning, or artificial intelligence. A clear strategic position helps companies to attract the right clients and foster strong partnerships.
Key elements of strategic positioning include:
- Understanding target demographics: Companies must comprehend who their clients are, what needs they have, and how they can best serve them.
- Value proposition: This entails articulating what makes their services unique and desirable. Developing a strong value proposition can lead to greater customer loyalty and higher success rates in contract acquisitions.
- Resource allocation: Firms should optimize resources based on their identified strategic position. Focusing on key strengths allows them to deliver better services and innovate effectively.
Companies that achieve a solid strategic position often enjoy several benefits. They can command premium pricing due to perceived superior quality. Moreover, they tend to experience reduced competition because other firms may find it hard to enter the same niche successfully.
In the data services landscape, understanding strategic positioning allows decision-makers to evaluate potential partners effectively. Companies with a clear focus can align their offerings with the evolving market demands, thus enhancing operational efficiency and market impact.
"Strategic positioning is not merely about being different; it is about being perceived as different in ways that matter to the consumer."
Competitive Advantage through Specialization
Specialization is a key driver of competitive advantage in data service firms. By focusing on a specific area of expertise, these companies can refine their processes and enhance service delivery.
Niche specialization allows for deeper expertise, which leads to improved quality and reliability. For instance, some businesses might choose to specialize in natural language processing while others can focus solely on image annotation.
This focused approach not only accelerates product development but also nurtures a reputation for excellence. Clients may gravitate towards firms known for their distinct capabilities. This enables companies to create more meaningful partnerships and enhance client relationships.
Specialized firms can also respond faster to market changes because they are usually more agile. This agility allows them to adapt their strategies in line with shifting industry trends or consumer demands. Industry players must recognize that while every service may seem necessary, those that offer specialized expertise will often lead the way in competitive markets.
Market Differentiation Strategies
Effective market differentiation strategies are essential for data service companies looking to establish and maintain a distinct presence. Differentiation goes beyond services offered; it encompasses the overall client experience, brand perception, and technological advancements deployed.
Here are several strategies that data service companies can adopt for market differentiation:
- Innovation in Service Delivery: Companies that embrace cutting-edge technologies often stand out. Investing in AI and machine learning tools can improve efficiency and accuracy, thus creating a superior service proposition.
- Customer-Centric Approach: Understanding customer needs and prioritizing their satisfaction fosters loyalty. Personalized services and tailored solutions can make a firm more appealing to potential clients.
- Brand Reputation and Trust: Establishing a strong brand reputation through transparent practices and reliability can set a company apart significantly. Testimonials, case studies, and consistent service delivery can forge trust.
- Collaborations and Partnerships: Strategic alliances with other tech companies can amplify market presence and broaden service offerings. This can lead to access to new technology, enhancing both service quality and customer satisfaction.
Customer Feedback and Satisfaction
Understanding customer feedback and satisfaction plays a critical role in the data annotation industry. Feedback acts as a bridge between service providers and clients, enabling companies to grasp client expectations and experiences. This understanding influences the direction of product development, enhances customer relations, and guides marketing strategies, ultimately increasing overall satisfaction.
Customer satisfaction is an indicator of a company’s performance. It reflects the degree to which services meet or exceed client expectations. High satisfaction levels can lead to increased customer loyalty, repeat business, and referrals. Conversely, if feedback is negative or highlights recurring issues, companies must evaluate their operational effectiveness.
- Benefits of Understanding Feedback:
- Improves product quality and service design.
- Helps in building a trustworthy brand image.
- Facilitates effective communication and relationship management with clients.
In a competitive field, leveraging customer insights can create a significant edge. It is not merely about collecting feedback but about analyzing it to derive actionable insights.
Analyzing Customer Reviews
Customer reviews can be a treasure trove of information. They provide direct insights from users about their experiences. Analyzing these reviews entails not just quantitative data from ratings but also qualitative feedback embedded in written comments. This analysis should focus on the following aspects:
- Common Themes: Identifying frequently mentioned features or concerns can guide product adjustments.
- Sentiment Analysis: Understanding the general sentiment behind reviews (positive, neutral, negative) allows companies to gauge public perception.
- Competitive Insights: By examining reviews of competitors, companies can spot gaps in the market or weaknesses in rivals’ offerings.
Tools such as Natural Language Processing (NLP) can automate the sentiment analysis of large datasets, making this process more efficient.
"Customer reviews provide critical insights into product performance and market perceptions."
Impact on Business Strategies
The feedback collected from customers significantly influences business strategies. Here is how feedback shapes various dimensions of business:
- Product Development: Continuous feedback ensures that products evolve based on user needs. This iterative process helps in refining features or adding new functionalities that resonate with client expectations.
- Marketing Strategies: Positive testimonials can be leveraged in marketing strategies to attract new clients. Conversely, understanding negative feedback helps in addressing concerns that potential clients may perceive.
- Operational Adjustments: Feedback often reveals systemic issues. By analyzing customer complaints, companies can streamline workflows or invest in training to improve service delivery.
Future Trends in Data Annotation Services
The landscape of data annotation services is evolving quickly. This evolution is shaped by the advancing technologies and changing market demands. Understanding these future trends is vital for organizations that rely on accurate and efficient data labeling for their AI initiatives. This section will delve into the predicted technological advances and anticipated market developments that are set to reshape the industry.
Predicted Technological Advances
Anticipated technological advances promise to revolutionize data annotation in several ways. New tools that leverage artificial intelligence and machine learning will drive automation in data-labeling processes. For instance, semantic segmentation and object detection algorithms can help minimize the manual effort needed for annotating images. This not only enhances efficiency but also ensures better accuracy in data quality.
In addition to automation, Natural Language Processing (NLP) technologies will improve the annotation of textual data. Systems capable of understanding context will produce more precise labels. Crowdsourcing platforms are starting to integrate these advancements, harnessing the power of machine learning to validate human contributions. Such validation processes can greatly reduce errors common in manually labeled datasets.
Another aspect to consider is the rise of real-time annotation tools. As machine learning models evolve, the need for rapid iteration will only increase. Real-time annotation can provide immediate feedback to model performance, enabling faster improvements. This is especially important for industries such as autonomous vehicles and medical imaging, where rapid decision-making is crucial.
Anticipated Market Developments
Market developments will also significantly influence the future of data annotation services. Increased partnership among tech firms is likely. Such collaborations will forge stronger integrations between data providers and AI developers. As data becomes more intricate, a synergy between diverse companies will enhance the collective capability to deliver better quality annotations.
Furthermore, as industries seek to implement AI solutions, demand for specialized annotation services will rise. Companies engaged in specific sectors such as healthcare, finance, or automotive may require annotations tailored to their unique data types. Therefore, companies providing niche services will have a competitive edge in positioning themselves within the market.
The regulatory environment is another factor to consider. As data privacy issues gain more prominence, companies in data annotation must navigate new laws and regulations. Compliance will be essential. Firms that can demonstrate strong data security and ethical practices in their annotation processes will appeal more to clients looking for trustworthy partnerships.
"The future of data annotation services hinges on adaptability to technological and market changes while ensuring data integrity and security."
In summary, being at the forefront of these trends will benefit firms engaged in data annotation services. Organizations that adapt to cutting-edge innovations while maintaining robust, ethical practices will emerge as the leaders in this evolving landscape.